AI & Energy Demands: Unlocking Data Center Efficiency
Ty Schmitt and Dr. Jeremy Kepner join David Nicholson to share their insights on the AI energy challenge, exploring the balance between innovation and sustainability.
The AI revolution has arrived, along with its massive energy appetite.
🌍 Six Five Media is joined by Dell Tech’sTy Schmitt and MIT’s Dr. Jeremy Kepner for this third installment of Dell’s AI & Us Series. Host David Nicholson and our guests explore the “AI Energy Challenge” and balancing the real energy demands of training and running powerful AI systems while creating innovative solutions for a more sustainable AI future.
💡 Key takeaways:
- AI’s energy needs are exploding, with some data centers consuming as much power as entire cities! (Think 60,000-80,000 homes!)
- While the news focuses on massive training facilities, even smaller AI applications contribute to the energy drain
- Collaboration is Critical: Breaking down silos between industry and academia in IT, facilities, and data science is essential for finding holistic solutions
- Liquid Cooling’s Rise: Liquid cooling is becoming increasingly necessary to handle the power density and cooling needs of AI
- The Human Factor: Policy and regulation in promoting sustainable AI practices and investing in people who understand computational science and performance engineering
Learn more at Dell Technologies and MIT Media Lab. Watch the full video above, and be sure to subscribe to our YouTube channel so you never miss an episode.
DavidNicholson:
Welcome to AI & Us, a series where we explore our future together in the age of artificial intelligence. In this episode, we’ll be exploring something we call the AI energy challenge. Specifically, what do CIOs and the rest of us need to understand about this subject? I’m Dave Nicholson with The Futurum Group. And I’m joined by two visionaries from MIT and Dell Technologies, both of whom stand at the leading edge of AI.
First, I want to welcome Dr. Jeremy Kepner from MIT. Dr. Kepner is a Lincoln Laboratory fellow and the head of Lincoln Laboratory’s Supercomputing Center at MIT. That supercomputing center happens to be the largest, I understand, in New England. Welcome, Dr. Kepner, and also Ty Schmitt from Dell. Mr. Schmitt is a Dell fellow within Dell’s Infrastructure Solutions Group. Welcome, I want to dive right into this, and actually starting with you, Dr. Kepner. Can you give us a quick summary of the current situation we find ourselves in? And where we’ve come from in terms of this power demand situation?
Dr.Jeremy Kepner:
Yeah. I think that the driving force, of course, has been AI. And AIs are powered by a particular technology called deep neural networks, which require enormous computation. And there’s two parts of that AI, both of which can cause a lot of power demand. When you build an AI, there’s a period called training where you’re taking enormous quantities of data and learning from that data to develop the AI, so that it can do its functions. And that can require an awful lot of computing power. Likewise, once you’re going to use the AI, that’s a period called inference. And that’s where you have lots and lots of customers out there logging into systems and trying to use the AI to answer questions. And both of them can be extraordinary, large consumers of energy.
David Nicholson:
And Ty, when we talk about extremely large, I threw out 150 terawatts, and we can all nod our heads and pretend like we know exactly what that means. But can you put some color around that? What is a lot of power?
Ty Schmitt:
I like to say that today we’re looking at an exponential amount of opportunities where these new, large opportunities are basically major cities worth of power. So historically, a large data center maybe 20, 30, maybe up to 50 megawatts in size, which is large. That’s basically table stakes for some of these large facilities today, we’re looking at 100, 300, 500 a gigawatt. And if you look at the average household consumer of power, multiply that out, you’re talking 60,000, 70,000, 80,000 homes of average power usage. So these are enormously power-consuming facilities for AI.
David Nicholson:
So if I’m in a restaurant in Boston and the lights suddenly dim, it would be right for me to shake my fist and curse Dr. Kepner’s supercomputing center, I guess. Is that what I’m hearing?
Ty Schmitt:
I don’t know. I would hope that the byproduct or the result of that supercomputer is maybe you get a better response on your order or your seating. Or maybe the meals that you’re getting come from a supply chain that was positively influenced by the machine.
Dr. Jeremy Kepner:
Well, I’ll just add in our case, our supercomputing center is really focused on science, and so does not have the tremendously high-availability requirements that you would see in industrial setting. So one of the methods that you can use to lower your power bill, is to say, “Look, we’re doing science. If we have a little blip here and there, that’s okay.” So we’re going to go down first so that the lights in your restaurant don’t dim. So that’s a classic trade-off people can do when they think about trying to lower their energy costs, is what is the availability that they need? In our case, obviously our systems don’t go down. But we invest in our own power backup systems, batteries and other types of things, so that we can ride through those types of demands. But with that, working with the local utilities, you can actually make it so that you’re the first ones to go down and give that load backup, so that critical services can maintain their capabilities.
Ty Schmitt:
When we talk about AI, everybody looks at the news and looks at, let’s say, some of the hype, some of the activity out there, and the requirements for net new power sources and things like that. The reality is is there are, relatively speaking, a small number of very large customers who are building and operating training facilities. And these are the major, call it, city’s worth of power that we’re talking about. The vast majority of customers, when we talk about inferencing types of workloads, these are smaller. And balancing resiliency, balancing performance, balancing the availability of power all factor into that.
David Nicholson:
So we’ve addressed the idea that the demands are skyrocketing for energy to power AI and other things in our lives. Ty, start with you, what are some of the best practices that you have seen put into practice? What should a CIO know about squeezing the bank for their AI watt?
Ty Schmitt:
Yeah, sure. I’ll say first of all, every customer I talk to, there’s a recurring theme. There are the constraints that they have today and whether they’re trying to maximize what they have, transform what they have, build something new, use different models. But inherently, we’re all challenged with the same aspects of the technology and balancing cost, time, performance. So behaviorally speaking, I think it’s critical that the preexisting silos between, call it, organizations or a company, facilities, real estate, IT, maybe data science. You have these areas that are solving their problems. The broader ecosystem, the broader solution, the best performance, the best value to come from that comes from breaking down those silo walls. I think there is more rapid acceleration in the advancement, the innovation of technology in the last two years associated with power and cooling, than I believe has happened in the previous, call it, 25 years. And that’s being driven by these awesome opportunities, these advancements in workloads, the productivity aspects. Ultimately, what is required to power and cool these machines. So you’re seeing more effective means of distributing power, monitoring power, controlling power, the same thing for cooling. And inherently, those come with efficiency. Everyone is trying to drive for the best performance per dollar, per set of constraints that you have, and so you’re inherently getting a lot of innovation out of that. I think really having the discrete areas of a company come together to understand and solve for the broader ecosystem is where that’s truly gained.
David Nicholson:
Very interesting. You didn’t immediately dive into liquid cooling. Everyone just needs liquid cooling, that’ll solve it. Obviously, it’s a more nuanced story.
TySchmitt:
Yeah. There’s a ton of constraints that are out there, so the investment required. So first of all, the path is to liquid cooling. It’s already happening, actually it’s been happening for a long time. Dell, we’re on our third or fourth generation of liquid cool solutions primarily for HPC. Liquid cooling is becoming more mainstream, and it’s absolutely required for the types of power density and cooling requirements that we see today and in front of us associated with AI. But depending on the customer usage model, they may not need it, they may not require it. And we have to recognize that and look at how do we optimize air cooling, liquid cooling, hybrid cooling to solve for those in the best possible manner? So it’s not all liquid cooling, but it’s heading that direction.
David Nicholson:
Yeah. Fair enough, fair enough. Dr. Kepner, Ty is going to provide us with the most efficient machinery to do the job that you throw at him. How are you going to make that job? What are your thoughts? How are you going to make that job more efficient from the start? Talk about, AI models and how efficiency might be driven there and whatever other thoughts you have.
Dr. Jeremy Kepner:
I’d say a big challenge is that the liquid cooling is evolving. And I think one of the areas that Dell is trying to really push forward on is getting a little standardization in the liquid cooling realm, which is really important for those of us who want to make long-term investments. We’d like to believe that we can invest in some liquid cooling capabilities that we can then reuse, and not have to replace them when we buy the computer. So I think that’s a really important area. With respect to the original question with, “Well, what can we do in the first place to make these AI models perhaps more energy efficient?”. There’s a lot of work right now on can we take these AIs and just make them very, very good at a particular problem? And then that can dramatically reduce the energy, essentially the size of the AI to something that’s much smaller.
Ty Schmitt:
With what is in front of us, the rapid pace of change, the transformation, I had two meetings with one customer out of Europe and another customer out of the UAE looking at new data center plans. And long gone is this aspect of a 25, 30-year asset that is designed to a design point, and then you begin to fill it up. So this need for looking at the entire lifecycle trying to project, we’re not going to get, if you try to plan for something 5, 10, 15 years out in this space, it’s probably not going to go well. What will go well is recognizing the rapid pace of change, the transformation that things may completely shift over time, building in flexibility into your system, into your design. And I would argue I’m a physical, mechanical-related person, but I think this probably applies to the software layer as well. But building that and that flexibility allows for options and change in lower OPEX, lower CAPEX associated with that in the future, it’ll pay dividends.
Dr. Jeremy Kepner:
I really appreciate that. And again, we saw a lot of folks who were building point solutions 10, 15 years ago, and they have to recapitalize those. And I think because it was a consortium that built ours, and we had a lot of really bright people who were helping with that, and people who think about the future of technology. And we were hearing what was going on in terms of the tremendous amount of power consumption and the new technologies that we’re going to require. We’ll pat ourselves on the back, but obviously we got a little bit lucky too, in that we did guess that right and built. And I think we did a really good job of making the flexible data center. I think for folks that are in much bigger scales, maybe that’s less because they can view an entire building as something that’s like, “Look, this is going to last five or 10 years.” And then we can optimize the whole building around a technology design point and perhaps get some savings, because you’re really thinking about that. But we have many, many, many generations of different types of technology in our data center, so it’s just a different design point.
Ty Schmitt:
Yeah. I think some of the classic MEP aspects of a facility, there’s a modular aspect of looking at logical building block sizes for the large power delivery pieces, even the cooling components. That really aligns well, in my opinion. And also in talking with customers of planning, having a master plan for whatever that future state is. But being able to consume and use based on chunk size optimization over time, and whether that’s two megawatts, five megawatts, 10 megawatts, whatever that may be. But allows for more of a, call it, aligned and optimized design point for near-term that improves time to operation and it reduces cost, it optimizes for OPEX. And then as things progress, allowing flexibility in the system to be able to either go higher, go lower, go sideways, whatever that may be at a future state. Instead of all that being incorporated into one monolithic system from a design point, is I consider very much a best practice.
David Nicholson:
So I love your speculation on where the power is going to come from, but mostly guidance for our fellow CIO types on how to move forward with this. Ty, why don’t we start with you?
Ty Schmitt:
Yeah, simple question, right?
David Nicholson:
Yeah, very simple.
Ty Schmitt:
So I tend to simplify where I can, call it a control statement, but if I look at load side versus line side. Load side being the workload, the line side being everything that is feeding that, and I’ll lump power and cooling and everything associated with that into that line side. Listen, it’s our job to maximize the performance per watt, performance per currency unit, and recognize the constraints, the flexibility that needs to go into to provide that. So in essence, basically maximizing how much performance you get per investment on the load side. The same thing needs to apply on the line side. So if we’re doing the right things to make the most out of the power that is being allocated for performance, that’s not just a statement of the IT equipment. That needs to propagate all the way through to the source power, and so part of that are having aligned KPIs. What are we talking about?
When we receive an RFP or a solution, are we aligned on the measurement points, what is actually critical and what are those tied to? If we’re not or if there’s confusion there that needs to be addressed. We all need to be on the same page of what is critical, what is going to be measured? And that drives all the behavior, I would say, both from a response standpoint, but more importantly, from an upfront investment standpoint. Having that information allows us to know where we need to be investing to improve efficiency, trade cost for feature set, things like that is absolutely critical.
David Nicholson:
What are your thoughts about what’s coming down the line?
Dr. Jeremy Kepner:
Well, I’d say that one of the things that we do a lot of, since we deal with folks who are mostly doing that training portion of AI, they’re developing models. They’re trying to create new models to solve new kinds of problems, and a lot of them are extremely knowledgeable scientists. We spend a lot of time though helping them become really good what we call computational scientists, in terms of figuring out how to get the most efficient and cost-effective usage. How can they make every single run of their model count, and deliver the most value and science for them? Because if you can do that more efficiently, well, it allows them to get their science done faster, which they love. It also just makes the system bigger for everyone. So there’s a lot of energy and efficiency to be gained by helping the people who are using it become more efficient. Likewise, on the macroscale with respect to energy itself, historically, one of the most efficient things you can do is put data centers next to energy sources. That’s been a thing that people have done for a very long time, not just in computing, but in other fields.
David Nicholson:
Very interesting. What is old is new again.
Ty Schmitt:
Well, I just wanted to build on the last couple of comments. First of all, I’m excited because the amount of innovation, the response from an industry standpoint, recognizing the opportunities to balance performance and cost efficiency, reduce carbon footprint in designs. Historically, the adoption rate for new technologies, whether it was driven by power cooling performance, it happened rapidly I think, relatively speaking. What we’re getting now is even much more rapid, accelerating curve there, and what it’s doing is it’s really fueling investment. It’s fueling investment in innovating and driving efficiencies, driving footprints smaller. Driving new design techniques that are basically providing the most value per watt provided, or square foot or whatever that may be. So there’s just more innovation occurring right now, and I’m just so happy to be in the middle of all of it.
And I think customers who are educating themselves, if I’m a CIO, understanding the broad aspects across my, call it, my cost centers, my company of where are we investing? How are we learning about these technologies? Because they’re not just points in time, they’re curves. We need to make sure that we’re connecting those dots and forming our own curves against our own constraints. The more progressive customers are doing that. And I know for our company, we’re helping customers as much as we can understand what those curves look like so that they can plan effectively.
Dr. Jeremy Kepner:
I think performance engineering is really one of the most valuable things that people can make human investments in. Companies having more people who understand these concepts, they just make your entire capabilities just run better.
David Nicholson:
You’ve seen these things, these data center cycles in the past, how has this change been the same?
Ty Schmitt:
Yeah. I’ve been at Dell for 31 years and was involved in owning our first through six generations from a power and cooling standpoint. Listen, 15, 20, 25 years ago, we were on a path to liquid cooling. The difficulty to power and cool was up and to the right. We were on a trend that was basically going to present real big problems from a technology standpoint in data centers. And what it did was it drove an awareness that, “Hey, if we can get smarter, better at how we design our products, how can we use sensors and inputs to control the speeds of fans to only consume the amount of airflow that needs to be consumed, so that I’m minimizing power?” Motor laws, the ratio of increase or decrease of a fan speed or a motor speed, or a rotation RPM, has a cubic effect on power. So there’s huge power gains by making small changes in motor speeds. Well, we recognized that and we said, “Hey, listen, if we can get smart about this and optimize our system design to only use the amount of airflow that we need, we can really drive power down.” And we did, and it did, and the entire industry did that. So we moved from a static design point to this dynamic design point of using logic, and control and feedback to get smarter. And all of a sudden, what was in front of us, I won’t say, got easier. We just innovated in a better way to solve that problem, and I see that happening today.
David Nicholson:
So if I’m hearing what you’re saying, intelligence can be applied to the very same machinery, if you will. And you can get much, much more efficiency without changing anything physically, just by monitoring and doing it intelligently.
Ty Schmitt:
It depends on the workload configuration, how it’s going to be used, but a high-power rack today is an order of magnitude or more than it was 15 years ago or even five years ago. So it’s happening fast. And what it’s doing is it’s driving the awareness to say, “If I can differentiate myself, if I can help solve for value for the customer. If I can help drive the things necessary to trade cost and performance in the right way, that’s going to be good for the customer, good for the earth, good for the environment, good for me.”
David Nicholson:
But Dr. Kepner, you’ve been around this for a while, is this completely unprecedented? Is it an acceleration? What are your thoughts with a historical perspective?
Dr. Jeremy Kepner:
Well, I think the really surprising thing that has been different, is we’ve been used to computing being important in our society and of growing importance. But the idea that it could be of the importance that we’re seeing now to unlock the investments that we’re seeing that are just extraordinary. The reason it’s kept going is because the scales of investment have just blown past what people felt were the limits of the past. That’s essentially what people are looking at is how can we apply these accelerators to a much broader range of problems, than the AI problems that we’re most interested in now? How can we apply them to discover new kinds of medicines? Or how to make new scientific discoveries, or how to build more durable systems? And these are just really exciting areas. And I’d say that’s the most exciting thing in science today, is this recognition that these AI technologies can be applied to a much wider range of problems that we would’ve ever imagined them being applied to even a decade or so ago.
David Nicholson:
Fantastic. Thanks to both of you, Dr. Jeremy Kepner from MIT, Ty Schmitt from Dell. We hope you found this conversation to be thought-provoking. The artificial intelligence journey is certainly just beginning, and we’re all truly in this together. It’s not just about technology. As you can see when we talk about energy, it’s about all societal inputs. And just remember that it truly is about AI & Us.
Other Categories
CYBERSECURITY
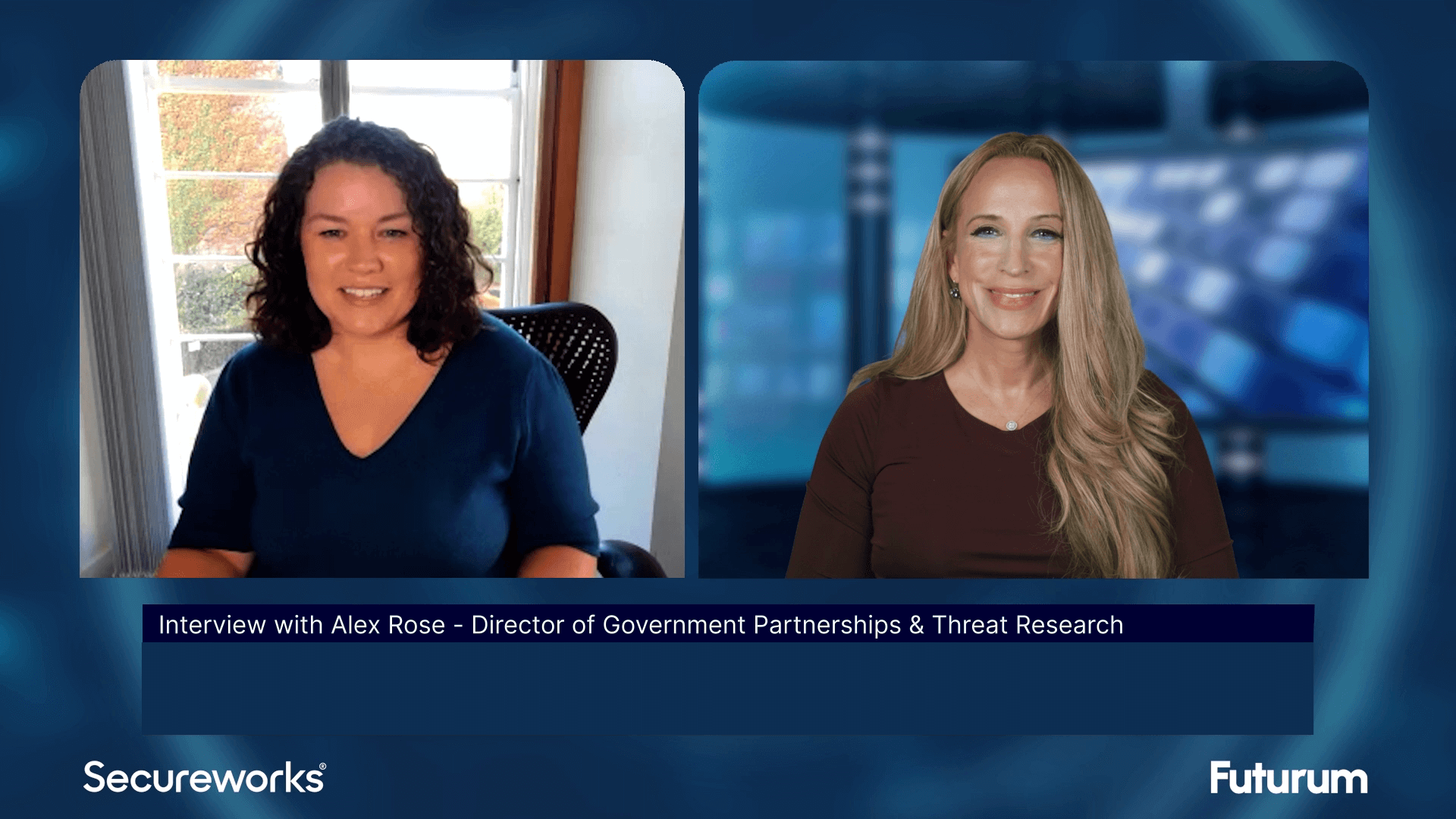
Threat Intelligence: Insights on Cybersecurity from Secureworks
Alex Rose from Secureworks joins Shira Rubinoff on the Cybersphere to share his insights on the critical role of threat intelligence in modern cybersecurity efforts, underscoring the importance of proactive, intelligence-driven defense mechanisms.
quantum
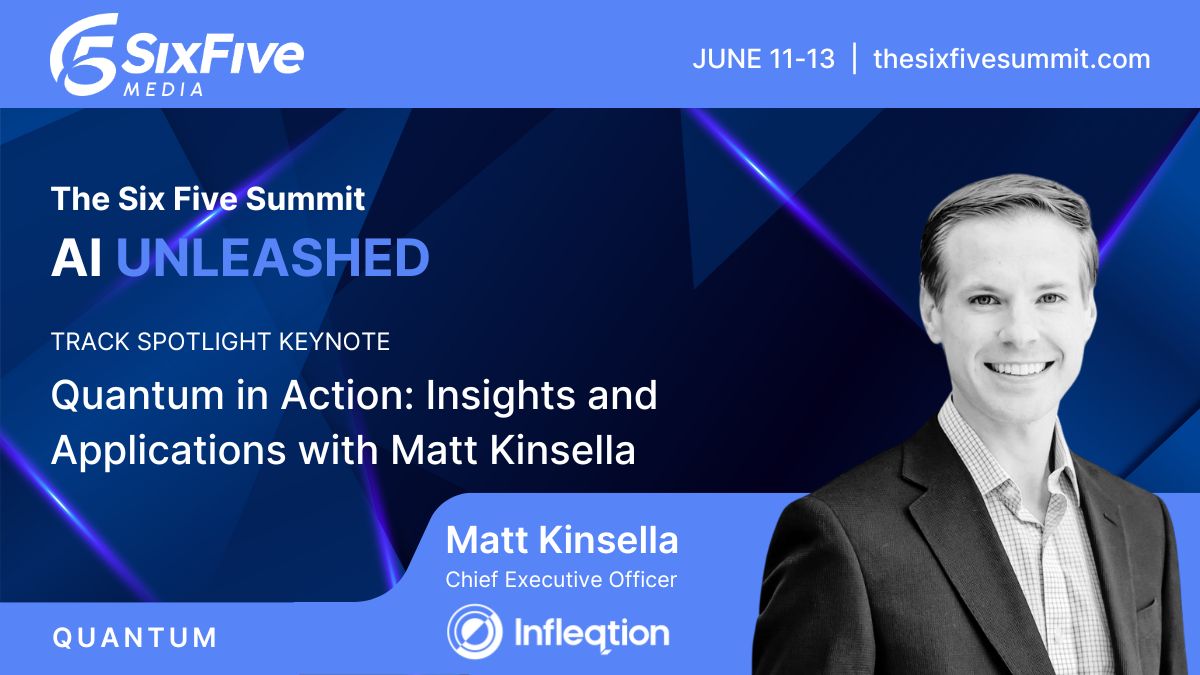
Quantum in Action: Insights and Applications with Matt Kinsella
Quantum is no longer a technology of the future; the quantum opportunity is here now. During this keynote conversation, Infleqtion CEO, Matt Kinsella will explore the latest quantum developments and how organizations can best leverage quantum to their advantage.

Accelerating Breakthrough Quantum Applications with Neutral Atoms
Our planet needs major breakthroughs for a more sustainable future and quantum computing promises to provide a path to new solutions in a variety of industry segments. This talk will explore what it takes for quantum computers to be able to solve these significant computational challenges, and will show that the timeline to addressing valuable applications may be sooner than previously thought.