Google's Workload Optimized Infrastructure at Next '25 - Six Five On The Road
Mark Lohmeyer, VP & GM Compute and ML Infrastructure at Google, joins the Six Five team to explore how Google Cloud's customized infrastructure meets the diverse needs of AI and cloud workloads.
The future of AI isn't just about the models; it's about the fiber, platforms, and systems that power them.⚡
At Google Cloud Next 2025, Patrick Moorhead and Daniel Newman are joined by Mark Lohmeyer, VP & GM Compute and ML Infrastructure at Google Cloud, to explore the crucial infrastructure driving the AI revolution and how Google Cloud is designing for the next generation of AI workloads. From system-level innovation across compute, storage, and networking to the unveiling of the AI Hypercomputer architecture, Google Cloud is facilitating accelerated AI training and serving through hardware!
Key takeaways include:
🔹Workload-Optimized Infrastructure: Google Cloud is laser-focused on delivering systems-level design across compute, storage, and networking, tailored to meet the unique demands of diverse AI workloads.
🔹Democratizing AI Access: Google Cloud's Vertex AI platform provides a user-friendly interface, enabling both sophisticated and enterprise customers to easily access and leverage Google's cutting-edge AI models.
🔹Driving Innovation Through Hardware: Google Cloud is investing heavily in a broad portfolio of accelerator platforms, including GPUs and TPUs, to provide customers with the flexibility and performance they need.
🔹Systems-Level Approach: Google Cloud is building a comprehensive AI hypercomputer, encompassing optimized hardware, software, and consumption models, to power the AI applications of the future.
Learn more at Google Cloud.
Watch the full video at Six Five Media, and be sure to subscribe to our YouTube channel, so you never miss an episode.
Or listen to the audio here:
Patrick Moorhead: The Six Five is On The Road here at Google Cloud next 2025 in my home amongst homes, Las Vegas, Nevada doing that tech thing. Been a great show so far. I mean a lot of talk about agents, of course in the context of Generative AI, but a lot of talk about what really makes the world go around is that software, is that infrastructure? The last two years has been infrastructure almost leading the way.
Daniel Newman: These things are all so interdependent, Pat. I mean we know that this build out of infrastructure is all about workloads, it's all about what can be consumed, what can drive productivity, what can drive efficiency gains. And of course we're seeing this era where it's sort of ushered in. We saw a very fast migration, it was machine learning and analytics and we were finally getting adapted to that. And then generative tools started coming out and now we, our sentences are being completed and our marketing is being written and now we have agents coming together and I think agents is really kind of bringing together the promise of automation, of intelligent process tools, of workflows, of generative and of course analytics all meeting and a heck of a lot of infrastructure is going to be required for that.
Patrick Moorhead: So let's dive in and talk about Google Cloud infrastructure. Mark, welcome back to the show.
Mark Lohmeyer: Thank you. It's great to be here.
Patrick Moorhead: Yeah, we were so pleased last year. We talked a lot about accelerators, we talked about CPU, GPU, all infrastructure. It was great. I think we all agreed that even though we always liked infrastructure, infrastructure is cooler than ever.
Mark Lohmeyer: Absolutely.
Daniel Newman: It was DPUs and XPUs and TPUs and there's going to be CP2, RPU. Whatever.
Mark Lohmeyer: Every possible copy.
Daniel Newman: I'm joking.
Patrick Moorhead: I might make up my own ship someday.
Daniel Newman: Why not just call you Chip from now on? So Mark, you heard our sort of entree into this conversation. A lot going on, you're focused on the infrastructure side of it, but it's all about being able to enable these other things that you're doing, whether it's air gapping Gemini or it's the workloads that are gonna enable agents to be successful. Talk a little bit about how you're kind of thinking about the infrastructure design to support the growing diversity of workloads around AI.
Mark Lohmeyer: Sure, absolutely. So you started with all these great examples of applications, right?
Daniel Newman: Here for you.
Mark Lohmeyer: And you know, thank you, and as an infrastructure person, you know, we like to think about this, our strategy as being a workload optimized infrastructure is what we're delivering. And the core idea there is we look at each and every workload or each and every application, and then we design at a systems level across compute, storage, network, hardware, software to meet the unique needs of each and every workload. And I think the thing that's fascinating right now is if you look at this next generation of AI workloads, mixture of experts, agents, agents working together, it's placing unprecedented demand on the infrastructure. And so we've been working hard to make sure that we've got the right capabilities to meet those next set of applications.
Patrick Moorhead: Right. So Mark, I'm trying to always try to put myself in the seat of the customer. And with all of these different workloads, with all of the different applications that sit on top of them, how do they know what infrastructure to use? I mean, okay, you have compute, but you have different storage, you have different networking, that all do things a little bit differently. It's one thing a sophisticated customer can probably figure it out, but not everybody can. And even if you're a sophisticated customer, how do I accelerate that learning?
Mark Lohmeyer: Absolutely. So I think looking at it from the customer's back is absolutely the right way to do it and the way we like to design our products. And you know, in simple terms, if you're an enterprise customer that's looking to maybe AI superpower an existing application or build a new app, you're probably going to want to do that through an API to a model as opposed to lower level access to the infrastructure. And for that we have a vertex AI platform. Right. So it makes it super easy. You've got the model garden, you have access to all the latest and greatest Google models, but you also have third party and open source models and you can easily integrate those with your application. You can tune them, you can ground them, and have days to go from an idea to actually something working, which is pretty amazing. But as you mentioned, there's also another class of customers that's maybe more technically sophisticated companies like Anthropic for example, or Hubex, that are basically building. Some of them are training their own models or tuning existing models, other of them are taking existing models and serving them at large scale. And those types of customers typically want to access the infrastructure at a lower level through something like Google Kubernetes engine for example. And then underneath that of course is the actual hardware, right? The GPUs, the TPUs, the CPUs. And from that perspective, I think one thing that sets Google apart is we have leading capabilities, leading choice of accelerator platforms, both for GPUs and for GPUs and CPUs, and we can maybe go a little bit deeper into that in a sec.
Daniel Newman: Yeah, it'd be a really interesting conversation I know many people want to have is they want to zero in a bit on, you know, why choosing a certain, you know, Pat and I, we're silicon geeks, we love semiconductors and of course we've seen how important they are to changing the world and everything. But, you know, if you maybe, you know, indulge us a little bit. We know you love all your children, meaning you love your merchant relationships, you love the silicon you're building, but really, like, what is the like kind of ethos as it pertains to Google about how you, you and your team and your sales are thinking about supplying the customer with the right silicon, giving them the flexibility they need to basically meet the, the demands, the growth, the expansion, all the things. Cause it's not just about the workload now, Mark, it's about where the workload is going to go and what the expectation is. And we all know how fast that's changing.
Mark Lohmeyer: Yeah, yeah, great question. So I think one of the things that makes Google unique here is that we operate at every level of that value chain, right? We're doing the research in Google DeepMind, we're building the models, we're serving those models in production in our consumer apps and then we're enabling the infrastructure that supports all of that. And so we're learning a lot from that whole process as well as from how we work with our external customers. And as part of that, we kind of get to see a glimpse of what the future is looking like.
Daniel Newman: Right.
Mark Lohmeyer: Or might look like, and then design that into the underlying infrastructure platforms. And I think the Ironwood 7th generation TPU platform that we launched here today is a fantastic example of that. We talk about that as a, you know, AI acceleration platform for the age of inference.
Patrick Moorhead: Right.
Mark Lohmeyer: Well, what does that mean? If you want to do fantastic inference, you need a lot of memory. You have to have the chips working together in unison to be able to serve these complex multimodal models with multiple agents communicating with each other all on the same platform. And so we looked at those trends at the application level and then we built the underlying capabilities into the TPU platform to support them.
Patrick Moorhead: Yeah, it really is a platform game for sure. I think, you know, I mean, just to be factual, you were the first ones to put together an AI platform. You surprised me last year when the research team said no, we train Gemini and TPUs and the world went nuts. Because wait, you know, how did, how is this done? How is this possible? And I think you reset. Well, first of all, you got a lot of credit for what you could do. And this isn't your first Rodeo. I mean, seventh generation on the TPU side, I guess 8 if you put, you know, 0.5, if that counts. Okay. But pretty impressive. And a complete “platformization” really is encompassed in your AI hypercomputer now, by the way, the first time when I heard it, I mean, I've done product management, I've done product marketing. I'm thinking about product marketing. Great, you know, great name there. But it's, it's a lot more than a name. What is it? What does it symbolize? Does it symbolize “platformization”? Does it symbolize the long term commitment you're making or something different?
Mark Lohmeyer: So I think that the core idea here is that it's really this integrated system that we're delivering to meet the unique needs of all of those different types of AI workloads and all of those different use cases, from training to tuning to serving. And we've designed and actually delivered this across compute, working together with storage, working together with the network at the hardware layer and then on top of that, optimized software. So for example, optimized support for Pytorch and Jax and things like VLLM on top of that underlying hardware and even one step further, making it easy for customers to consume it commercially with things like dynamic workload scheduler that allow you to say, hey, I need a certain amount of accelerators for a certain period of time, you know, two weeks from now.
Patrick Moorhead: Right.
Mark Lohmeyer: Guarantee they're gonna be there when you need them and then only pay for them when you're actually using them. So it's really this systems level design across hardware, software and consumption models that's at the heart of the AI hypercomputer now.
Patrick Moorhead: I appreciate that.
Daniel Newman: So I want to go back a second to talking about Vsav and Ironwood. You mentioned inference and you know, it was kind of, as you were talking about it, it just kind of dawned on me. We know that inference is going to be this sort of parabolic growth, right. If you just look at agents, for instance, right. We're still in this kind of very new era, a new dawn of this, where you have companies deploying a few agents. We haven't gotten to the point yet where you have thousands and now millions and eventually probably billions and trillions of these things working. So inference becomes the killer workload. But obviously you have to think about that because there is a lot of training, there's test time, there's, you know, all the different ways that you're going to basically tune models. You talk about the platform, you talk about this being inference centric. Are you going to still focus on training? Pat mentioned the training of Gemini. Are you guys sort of looking and saying maybe we want to double down on where we see the market going and not be so is there any sort of thinking to that or is that just where you landed on Ironwood?
Mark Lohmeyer: So it's definitely for both. And so training is a significant investment for Google. It's also a significant investment for many of our customers. We expect that to continue to grow and we're continuing to invest in that. For example, we recently enabled a software capability called cluster director that allows you to treat a large number of individual accelerators in a cluster as a single logical entity. So deploy them, manage them, predict failures before they might occur and actually correct them before it causes an issue with your training job, ultimately shrinking the time to do a training of a large model down significantly. So we're continuing to invest full steam ahead and training. Now that being said, to your point exactly, you know, 2025 is the year of inference, right? We're already seeing it going through the roof from a Google perspective. We're seeing huge interest from our customers and so that's the next big area of focus. But we're going to definitely, definitely do both.
Daniel Newman: No, I think that's great. I think it's just one of those particular areas where we know that there's a lot of companies trying to inference and then you're even seeing kind of layering down to like we're only trying to do video or we're building chips that are only for edge or we're building chips that are only for. So there is still a lot of processing, kind of what platform, what architecture, what's gonna kind of lead. And by the way, I think Pat, you and I both say this frequently, but this kind of zero sum mentality that tends to exist. I like that Google has sort of the, we love our merchants, we love to build and make sure we create what's custom for us. Because I think in the end the market's so big, whether you have the head half trillion dollar number by the end of the decade or the trillion dollar number, it's like do we really have to settle that there's only one company or two like there can probably be 10 or 15. And by the way, we know from the CPU era that it's probably best for the market to have some choice. And I think that's something that we've hopefully gone back and we learn from what's happened in the past to make sure that we stay on the cutting edge of innovation.
Mark Lohmeyer: Yeah, absolutely, absolutely. And I think a lot of that initial value we think we can deliver is actually in the software on top of the hardware. Right. And so if you think about customers wanting choice and flexibility, yeah, they want to be able to sometimes use GPUs, other times TPUs, other times CPUs, but they would like to have them all operate in a consistent way. They'd like to have the models be able to easily move across those different underlying hardware platforms without having to re optimize everything. And so we're investing a lot in the software layer on top that makes that possible. And I'll give you one specific example of that that's super exciting to a lot of customers for inference. So there is an open source inference engine called VLLM, and it's getting huge traction in the market. It got its start with Pytorch on top of GPUs, and that's fantastic. We support it there. But then today we announced that we're also extending support for VLLM with PyTorch on TPU's. Right. And so now it makes it super easy for a customer to run the model across either of those two platforms as they choose.
Patrick Moorhead: Well, in the end, enterprises want choice, they want optionality. And while in the beginning of a cycle there might be a, you know, a rabbit or something that gets out, but, but in the end they want choice. And it just gives it the ability to sleep well at night and understand that they're not connected to just one method, just one vendor. And I'm really happy to hear about the VLLM support.
Daniel Newman: Yeah, we're definitely hearing a lot about that, Mark. And we're hearing a lot about sort of the abstractions moving higher up and VLLM sort of opening up the gambit to different, you know, different frameworks being able to basically write software to different hardware architectures. And I think that's when you really start to get the scale with a, and the scale with, you know, the future of AI's potential. Because you all know, while we're loving this CapEx moment, we're loving this build out. I think the world really wants to know when we start to see it consume, we start to see it grow. We start to see it change industries and really drive the next wave of productivity. Well, Mark, I want to thank you so much for joining us here on The Six Five. It's been great to chat to you at Google Cloud. We seem to do this on an annual cadence so if we don't do it before, let's be sure to do it again next year.
Mark Lohmeyer: Absolutely. Always enjoy the discussion and look forward to it.
Daniel Newman: Thank you everybody for being part of The Six Five. We are on the road here at Google Cloud Next 2025 in Pat's second home Las Vegas, Nevada. Hit Subscribe. Be part of our community. We appreciate you tuning in. Check all our coverage out here at Google Cloud next and of course join Pat and I each and every week for our Six Five where I win all the debates. Talk soon. Bye.
Other Categories
CYBERSECURITY
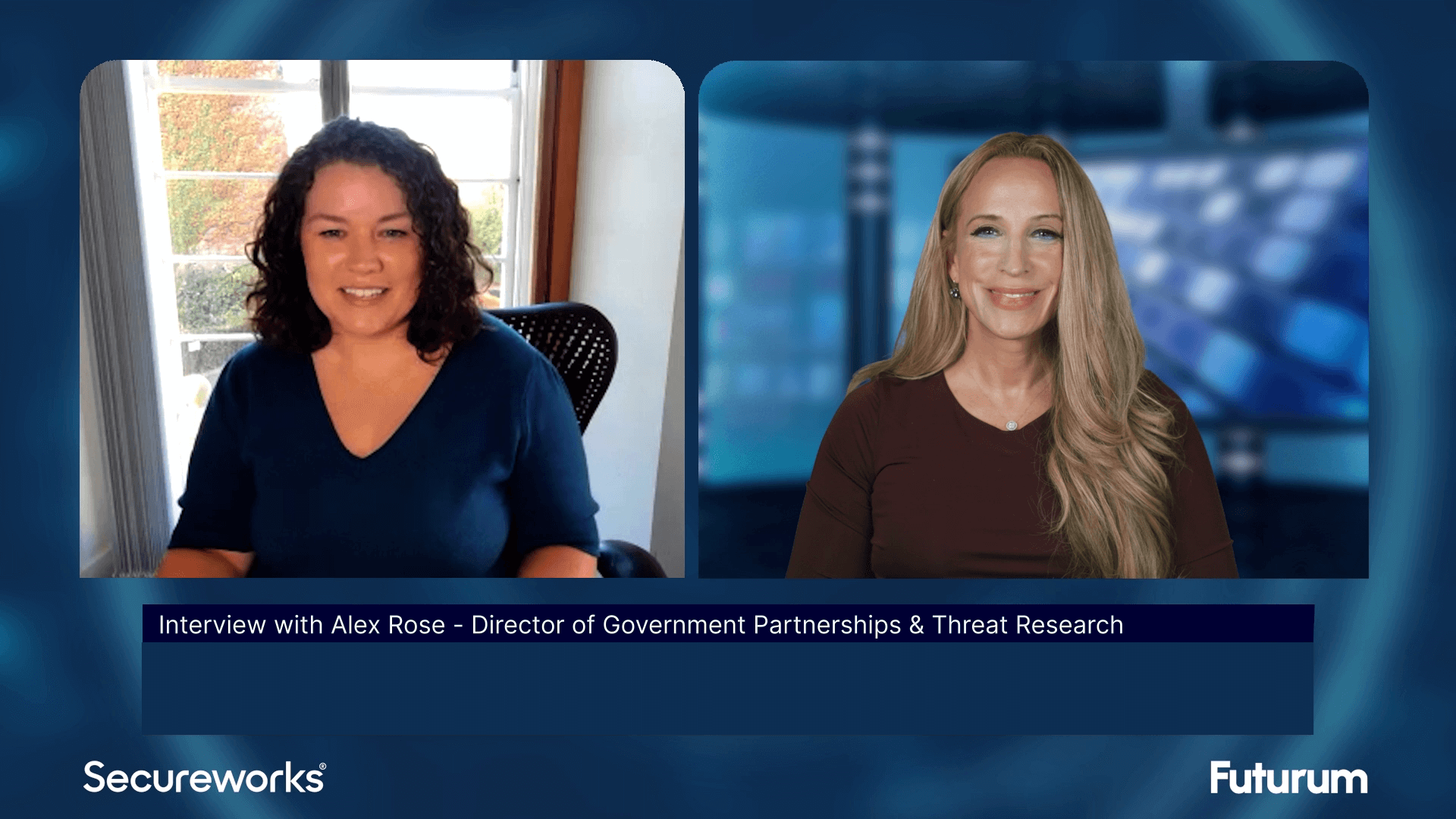
Threat Intelligence: Insights on Cybersecurity from Secureworks
Alex Rose from Secureworks joins Shira Rubinoff on the Cybersphere to share his insights on the critical role of threat intelligence in modern cybersecurity efforts, underscoring the importance of proactive, intelligence-driven defense mechanisms.
quantum
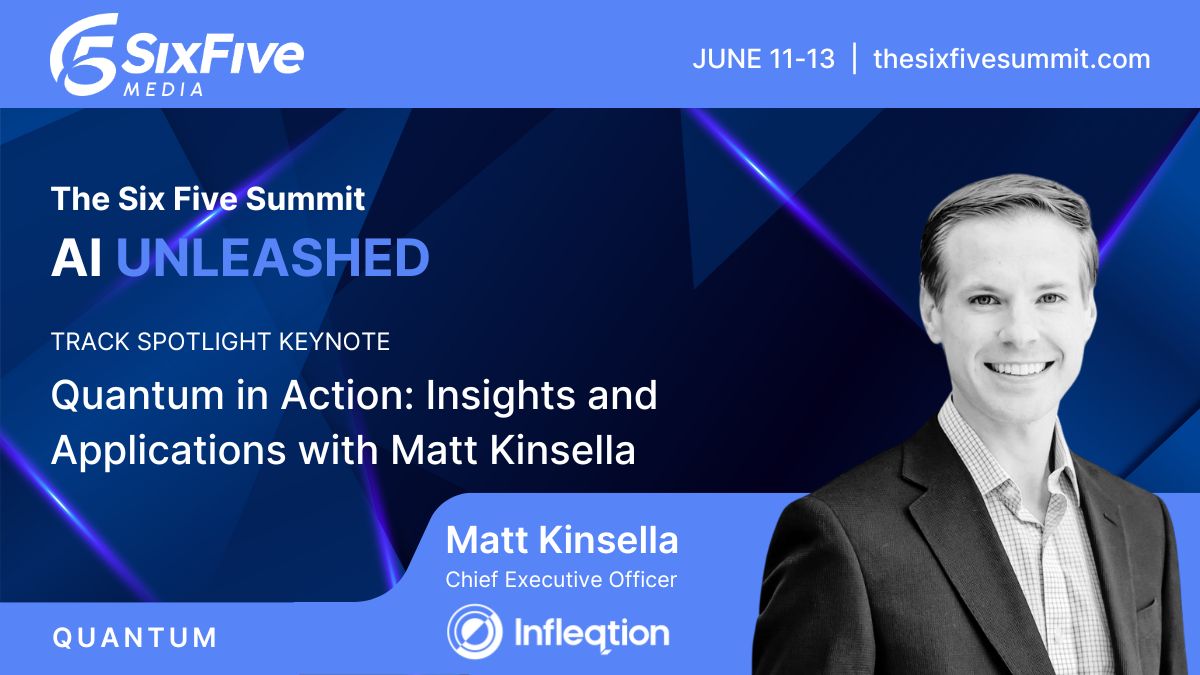
Quantum in Action: Insights and Applications with Matt Kinsella
Quantum is no longer a technology of the future; the quantum opportunity is here now. During this keynote conversation, Infleqtion CEO, Matt Kinsella will explore the latest quantum developments and how organizations can best leverage quantum to their advantage.

Accelerating Breakthrough Quantum Applications with Neutral Atoms
Our planet needs major breakthroughs for a more sustainable future and quantum computing promises to provide a path to new solutions in a variety of industry segments. This talk will explore what it takes for quantum computers to be able to solve these significant computational challenges, and will show that the timeline to addressing valuable applications may be sooner than previously thought.