Home
From Proof of Concept to Production: How AWS re:Invent 2024 Strategic Partners are Shaping Enterprise IT
From Proof of Concept to Production: How AWS re:Invent 2024 Strategic Partners are Shaping Enterprise IT
Baskar Sridharan, Vice President at Amazon Web Services, shares his insights on transitioning generative AI from proof of concept to production, emphasizing AWS's role in shaping the future of enterprise IT.
Generative AI is no longer just a playground for tech enthusiasts. Hosts Daniel Newman and Patrick Moorhead are joined by Amazon Web Services' Vice President, AI/ML Services & Infrastructure, Baskar Sridharan on this episode of Six Five On The Road at AWS re:Invent. They discuss the journey from proof-of-concept to full-scale production in enterprise IT with a focus on generative AI and strategic partnerships.
Highlights Include:
- Great Expectations: The transition of generative AI applications from experimental stages to production and the evolving customer expectations of AI & data infrastructure
- Unified SageMaker: AWS is streamlining the journey from data to AI with their next-gen SageMaker platform, making it easier for businesses to build and deploy GenAI applications
- Cost Optimization: Model distillation and other innovations are making GenAI more affordable, with significant reductions in training and inference costs
- Data as a Differentiator: Your data is what makes your GenAI applications unique & AWS is providing powerful tools like Bedrock Knowledge Bases to help customers leverage data effectively
- Trust and Security: AWS is leading the way in responsible AI with its ISO 42001 certification, ensuring that your GenAI applications are built on a foundation of trust
- Real-world examples: How enterprise IT is leveraging AWS services to scale their generative AI applications effectively
Learn more at Amazon Web Services. Watch the video below, and be sure to subscribe to our YouTube channel, so you never miss an episode.
Patrick Moorhead:
The Six Five is On The Road here in Las Vegas at AWS re:
Invent 2024. It should be no surprise that most of the announcements are about AI, specifically generative AI. Daniel, great event so far.
Daniel Newman:
It’s great to be here, and we’ve heard a lot about moving the needle and that the needle is going to be moved. And so far what I’m seeing is a lot of focus on AI, but not just on AI as a whole. I think we’re turning the corner, Pat, from this Rev-1, Gen-1 that started a couple years ago, and now we’re really into this finding value from AI. And that’s really the feeling I’ve gotten so far during our time here at re:
Invent.
Patrick Moorhead:
For sure. And listen, the tech is cool. We love tech, new tech, but the reality is for enterprises and businesses to adopt this, you need to have the right tools. They need to be simple. I mean, nothing is simple in tech, but as simple as they possibly can be. And there were some investments done in that old AI, the machine learning stuff, that needs to be capitalized and used as well. And I can’t think of a better person to have this discussion. Baskar, welcome to The Six Five.
Baskar Sridharan:
Thank you.
Patrick Moorhead:
First time guest. This is wonderful.
Baskar Sridharan:
Absolutely. I’m excited to be here.
Patrick Moorhead:
Yeah, congratulations on all the announcements.
Baskar Sridharan:
Thank you. This has been absolutely a great set of few days. It’s probably one of the most exciting set of launches we have actually done on GenAI and data and ML and how we are bringing all of them together. So I’m super excited to be talking about those with you folks.
Daniel Newman:
Yeah, it was a really exciting run up. There was a lot of press, a lot of speculation about what’s going to happen. You’re the largest infrastructure provider in the world and, of course, one of the most exciting companies that really fundamentally changed compute and computing and basically enterprise access to the cloud. And so now there’s so much focus, you heard us in the run up, Baskar, but there’s so much run up as to how do we get value, how do we start to put some… We’re beyond the whole just CapEx spending and standing up GPUs. And now people are starting to talk about, okay, we’ve got all this data. We have these AI tools that we’ve picked and we’re investing in. We have stuff that’s openly available. We have stuff that’s proprietary. You’re seeing this handoff, data, AI, tools. What are some of your recommendations to your large set of customers about how to make this handoff streamlined for efficiency and to really move AI projects forward?
Baskar Sridharan:
Yeah, it’s a great question. I think it’s a perfect tee up. This is one of the reasons why we actually launched the next generation of SageMaker. We have been hearing from our customers how difficult it is, like you rightly mentioned, to actually get value out of disparate set of data sources that they have. You spoke about how customers are generating their own ML models there, and then now they’re wondering how to actually link them all both to get to a GenAI app development. So this whole process from moving from data to analytics to ML to GenAI has actually been a very siloed and disparate set of experience to the customers. And we have been hearing this from our customers in terms of how we can actually bring them all together.
And that actually led us to the announcement of the next generation of SageMaker where we provide a seamless way to move from a disparate set of data sources and being able to do data processing on them, being able to actually take the data that is actually in Redshift or in S3 or in a federated data sources and being able to do SQL analytics on it, and then connect them all to your ML model development and then actually build a GenAI app on top. So being able to move from data to analytics to ML to AI, we have made it super easy with the launch of the next generation of SageMaker. Now, all of this is probably not that useful if you don’t have the right security built in. So one of the things that we focused on is how do you actually ensure that it’s uniform governance, uniform way to regulate access to the data, access to the models, and then being able to tie them to the entire development lifecycle from data to ML to AI.
Patrick Moorhead:
No, that’s good stuff, and I’m glad you’re hitting on data. I mean, two years ago before it was cool and people started talking about what you could do with generative AI and LLMs and the ability to take data sources that were typically disparate, your CRM, your ERP, your PLM, all these fancy acronyms for enterprise apps, and that changes the game as well. And I’m sure as you’ve seen, and I’m pretty sure I’ve heard you talk about, is the requirements of moving from I’ll call it science project to POC to scale for the generative AI scaffolding and tools and database and data management, it changes. Is that correct? Can you talk me through that? How has it changed? How have your customers expressed the need for a different type of capability once they’re scaling?
Baskar Sridharan:
That’s a great question. I think if I look at the vantage point, the last 18, 24 months I think have predominantly been about how do I actually try a bunch of prototypes for GenAI to get value. So it’s been unprecedented way of exploration of prototypes. And that’s what I call as act one, how do you move from act one to act two where you’re now taking some of these prototypes and putting them into production, but now you want to be able to do this in a more secure, scalable, trustworthy manner and you need to be able to build a viable business that’s actually scalable. How do you do that? And this is where I think our announcements for the next generation of SageMaker is actually extremely compelling.
So if you take data processing, we have Amazon Athena, we have Amazon Glue, we have Amazon EMR, we have Amazon Managed Workflow for Apache Airflow, all of that helps you prepare your data and use the data for orchestrating and building your GenAI applications. Now, if you move to SQL analytics, we have integrated Redshift with SageMaker Lakehouse. And so now the data that resides in Redshift are the data that actually resides in S3 or in federated data sources, like you said, in ERP and other places. You can now bring them all together and have a single way to govern and a single way to look at lineage across all of these and then do SQL analytics on top. Now, all of this is useful only if four conditions I think are true. One I think is data quality, garbage in, garbage out.
Patrick Moorhead:
Sure. It’s been that way for a long time. Forever.
Baskar Sridharan:
And that’s true in GenAI too.
Patrick Moorhead:
Amplified in GenAI.
Baskar Sridharan:
Absolutely amplified in GenAI because you want to be able to provide fresh contextual data. So data quality. Second is when I spoke about prototyping, customers are mostly in silos. So when you’re in silos, you can use silo data sources and you can create your own prototypes. But now when you want to go into production, you have to find a way to unify all of these data sources together and find a single way to govern these data sources for your GenAI application.
How do you do that? So data governance is the second piece. The third piece is security. You want to be able to have the right level of security across this diverse set of data sources and integrate them into your development lifecycle. The fourth one, I think you touched upon it, which is how do you provide the right infrastructure and tooling to be able to do this at scale? And this is one of the things that AWS absolutely excels in, which is providing all of these sources of tools that can actually help you scale with the various data sources that you have underneath the course.
Daniel Newman:
It’s interesting. We all, I think at some point in this brief conversation, referenced the two years. And of course, since ChatGPT. That was like this weird inflection that sits on a continuum of AI. And of course, people like you that have been around SageMaker for a while know that ML has been a much bigger part for enterprises for a long time. And of course, what, you’ve used this stab at 40, 50 years since the earliest algorithm. So AI is not new, and I think everybody out there needs that reminder at times. But what we are seeing now is customers… You said act two, so you’re a theater guy. You appreciate the theater.
But what we are seeing is expectations change. Two years ago, it was getting on earnings calls and everybody’s seeing how many they could say AI, and you got value in your stock for that. Now I think there’s a new level of accountability being held. And that new level of accountability is how are you deriving value and actually being able to express that through customer experience, employee retention, product development, and innovation. So your customer’s expectations from two years ago when you started with Bedrock, next generation, they’re evolving. Can you talk a little bit about that evolution, what they’re seeing, what they’re asking for, what they’re expecting to be able to really meet that act two that you just…
Baskar Sridharan:
Absolutely. Absolutely. I think the act two, which is essentially how do I take some of these prototypes and put them into production, requires customers to look more critically in terms of how do we actually make it cost-effective for not just training purpose, but for also inference purpose? So one of the key things that we look at when we actually look at what needs to get into production, I would say there are probably four key drivers. First I think is cost. How do you actually control the training cost? How do you control the entrance cost? And we announced a bunch of new features in the last few days, which spoke about how do you actually reduce the training costs? So Amazon SageMaker, for example, we actually said, hey, we have a way for you to reduce the training costs because we can now create flexible training plans for you.
We can actually go on the tasks for you automatically, and we can better use the compute resources that are actually underneath the covers. How do you make them more efficient? So these actually reduce the cost for training. Now you’ve created a model or you’ve basically chosen one of the models that are publicly available. How do you now reduce the inference cost at runtime? That becomes another important issue when customers go into what I call as act two. And here, Model Distillation, that we announced recently, is an extremely capable way by which you can take a publicly available foundation model and then make this big model, which is a teacher model, teach a smaller model that is actually more cost-efficient, more performant, and actually is more accurate because of the data that’s been trained with, which is your company’s specific data.
That takes care of cost. Now you have to come to model choices. Now, models are available to everybody. Foundation models are available to everybody. So what is the differentiator when you actually want to move from prototype to production? The data becomes the differentiator to your point. The data sources that you have become the differentiator. And how do you take the data and unify that with the model so that your generic application is not a generic application, but it’s actually something that reflects your company’s culture, your company’s values, and your company’s documents. So the releases that we did on Bedrock Knowledge Bases, the graph frags, they actually help you take data that’s specific to your organization and actually combine it with the models.
And the last one I think is trust. How do you actually go build trusted GenAI applications? And how do you instill trust not just in the application, but also in the users of the application? And we announced that Amazon is the first public company, cloud company, which is ISO-42001 certified. And this certification essentially is a public certification that allows Amazon and the scrutiny of how we actually develop these AI systems and what that actually means for instilling trust in our customers.
Patrick Moorhead:
Wow! A lot going on here.
Baskar Sridharan:
Lots going on here.
Patrick Moorhead:
Baskar, I’m a recovering product person industry guy. So I used to do that. And now I run an analyst company. I get to go to all the announcements. So it’s like heaven for me. This is great. And one thing that I really appreciate about AWS is you’re a product company and go through, here’s the customer problem, here’s the pain points it’s causing, here is what we’re bringing to market, here’s the solution, here’s how it works, and here’s the benefit. I love that, by the way. But I have to ask you, you did hundreds of announcements here. I’m going to have to ask you, which one of your generative AI product children gets you most excited?
Baskar Sridharan:
Fantastic. You’re asking me to choose between each of my…
Daniel Newman:
I love all my kids the same.
Patrick Moorhead:
I know it’s tough, but the problem is you can’t list a hundred. We’re going to limit you here. What are your favorite or the ones that get you most excited?
Baskar Sridharan:
Let me walk you through what I think are the logical layers of how customers would interact with AWS services than the ones we announced. At the outset, as I mentioned, I’m really excited about the Unified SageMaker, the next generation of SageMaker that we announced, because this now truly addresses a key customer ask, which is please help us move from data to analytics to ML to AI in a more seamless and integrated manner. And I’m super excited about that announcement. Second I think is if you come to Amazon Bedrock, we spoke about how cost is very critical for inference, so we announced Model Distillation. And here you can take a teacher model and you can use that to teach a smaller model, which is more efficient at cost, more and more accurate and faster too. So cost, accuracy, and latency, all three are better with Model Distillation.
And in fact, models that are distilled using Bedrock are a whopping 500% faster and they cost you 75% cheaper to run. That’s pretty significant when you’re looking at running inference at scale. Second, if you look at the work that we did on multi-agent collaboration, now, one of the things that I think was a transition point between companies moving from prototype to production is the realization that one model is not going to rule them all. So how do you build these siloed interfaces where an agent is actually good at one specific task, you now create a bunch of these agents and now you want to have a leader agent kind of, which will coordinate across these agents to produce the right response to the customer?
So we introduced multi-agent collaboration, which allows companies to be able to build these agents and deploy them into production and collaborate across these agents to produce a single unified response. On Amazon Bedrock, we introduced Bedrock Marketplace, which provides more than 100 plus models that are available. These are diverse models, so customers can pick and choose which model they want for their particular use case. And finally, on Bedrock, again, I didn’t want to list the entire list, but if I have to choose my top few children that I love…
Daniel Newman:
Many children. He loves them all.
Baskar Sridharan:
Exactly. The Bedrock automation, data automation, this is very critical. We were speaking about this earlier. Companies have a diverse set of unstructured data. Some of them are PDFs. They’re images, audio, and video. So how do you take this large collection of diverse unstructured data, very easily transform it using a single unified API so that you can now create value and insights from this data? So the Bedrock data automation is something I’m very excited about because it brings in this capability at scale that will help you get to act two. On the SageMaker AI piece, I think there are three things I would love to highlight, the ones that we announced a few years ago. One is the task governance piece. One of the things that companies struggle with is how do you manage the training tasks and can Amazon help govern and automate this task for the customers?
So the Amazon SageMaker AI task governance, it helps customers achieve more from their compute resources than otherwise. Second is we also introduced flexible training plans. So now you can just specify your budget, you can specify your timeline, and you can specify how much compute resources you need, and we will create, Amazon SageMaker HyperPod will create an automated training plan that suits your budget and your constraints. And we also introduce recipes. So customers who want to create very easy way for them to fine tune and train a publicly available model, we make it super easy for them to be able to do that. And we also introduced partner apps as a part of the SageMaker Studio where you can now easily use partner apps inside your SageMaker HyperPod, instead of having to go build your own managed instances of these services. So I’m super excited about some of these things. I could go on and on, but as you said, I have to stop. It’s some of my favorite ones.
Patrick Moorhead:
We’ll make it tough.
Daniel Newman:
We’re going to make you choose before this ends up being an episode of Rogan or a three-hour-long podcast in here. But by the way, it sounds like we’ve got the material, so maybe we’ll have to plan that later, Baskar. Let’s get a little practical here. I agree with you. Most of the consumption is not going to be… There’s a handful of companies, including Amazon, they’re going to do the training. They’re going to build models. For the rest of us, it’s going to be techniques. It’s going to be fine-tuning models. It’s going to be RAG. It’s going to be small language coupled with these larger models. Can you share maybe some examples of customers that are building on these services and what are their outcomes? Is there any specific customers or even generic customer opportunities?
Baskar Sridharan:
Yeah, fantastic question. I think as you saw in my talk, we have DoorDash. So DoorDash has actually built on top of Amazon Bedrock and Knowledge Bases and Amazon Lex. So they have created a wise conversational AI agent that can actually respond with low latency when what they call as dashers have a question. And so they have used Amazon’s Knowledge Bases to create the actual rate response data source. They have used Amazon’s Bedrock for the choice of LLMs, and then they have used Amazon Lex for wise conversational AI. So putting them all together, they have created an actual conversational agent that’s actually used by the dashers in real time, hundreds of thousands of times a day. And I found it extremely impressive to see a real world application of these agents being built in production.
And as you might have seen, we also had Phil Mui from Salesforce, and they demonstrated how their Agentforce, which is that autonomous agents piece, and they have the Atlas reasoning engine, which is all built on AWS, that’s, again, another example of customers now running production workloads on top of AWS’ GenAI services. In fact, I think Salesforce’s data cloud and also the Einstein Studio, they now expose the Bedrock models for their own customers. In fact, Salesforce’s research team uses SageMaker HyperPod to develop with the models and actually use the SageMaker’s inference components for actually using their models for inference. So we have a lot of these very large-scale deployments of GenAI that’s already happening. And it’s super exciting for me to see that customers are truly moving from act one to act two. And as I mentioned in my talk, Gartner predicts that only 30% of these prototypes actually move into production. So to see that these companies are actually in production at scale is extremely exciting.
Patrick Moorhead:
So just to keep the trend here of practicality, and I think you addressed it a bit when you were rolling out the new tools and capabilities, but what would be some key areas that enterprise should focus on moving from these POCs into production? What do they need to be thinking about most?
Baskar Sridharan:
Great question. I think there are four drivers. First is models. How do you choose your model? And you need a choice of models there. And with AWS, we have a choice of models through not just the curated models in Amazon Bedrock, but we also have the Bedrock Marketplace where we have over a hundred models. We also have the custom model import where customers can take a publicly available model architecture and customize them and then import them into Bedrock. So I think the models and the choice of models is pretty critical. And I think as I mentioned, there is no single model to rule them all. The models have been leapfrogging one over another. And so you want to be able to build your application and not have to change it every time you choose to run on a different model. So that’s number one.
Number two, I think, is cost. I think we covered that, which is how do you manage not just the training cost, but also the inference cost? And what are the tools that AWS can actually offer in terms of distillation, fine-tuning, and prompt caching? And we announced a bunch of these in re:
Invent this week, and using them to reduce your inference cost and manage both the training inference costs across the board. The third one I think is data. We spoke about it. Data is the lifeblood of any GenAI company. And in fact, I would say that your customer’s data is the differentiator between a generic AI application and one that’s customized to respond to your customer with your company’s information and with your company’s culture.
And the work that we have done on the next generation SageMaker and the Bedrock guardrails and Knowledge Bases announcements on GraphRAG and Knowledge Bases evaluation, all of them, LLM as a judge, they all actually help you do this, which is take the data that’s your differentiator and integrate them into your application. The last one, but not the least, is the trust. And as I mentioned, AWS is the first large cloud provider to be ISO-42001 certified. And we truly take it seriously in terms of how we think about security and trust when we help our customers build these into the application so that they can now use that to earn trust from their users and their customers. So these four, I think, are pretty key drivers when it comes to GenAI development.
Patrick Moorhead:
Sage Words. SageMaker, Sage Words, you get that?
Daniel Newman:
Nice job.
Patrick Moorhead:
Thank you.
Daniel Newman:
So there’s a lot of here. And personally I have to say, very excited about the API that enables all those structured and unstructured data sets to be utilized. I think unlocking the enterprise data is still more challenging. We’ve been talking about this. There was a decade of big data before generative AI that we were talking about getting your data estate right, and that was still a problem as we ran into generative AI. I also really love what you’re doing on the Agentic side. Interesting to see what you and Salesforce are putting together, combat some of the… Because one of the things that sometimes people will say about AWS is not as app focused, but the way that you can actually become a great partner to some of the leaders in apps, I think this opens the door for you to be the partner of choices.
Baskar Sridharan:
Absolutely.
Daniel Newman:
That’s a great example of Salesforce. Let’s end on something a bit more of a personal anecdote. So obviously you’re at the forefront of a very important transformation that’s going on. You’re driving development. You’re making choices. We know that the leaders in this space right now are thinking about data utilization. They’re thinking about ethics. They’re thinking about security. They’re thinking about obviously growth and economic productivity, efficiency. Talk about your journey, your experiences that have shaped how you’re thinking about all this and how you’re driving your product org.
Baskar Sridharan:
Yeah, it’s a great question. I think one of the things that I would say, it’s probably a personal anecdote, but I think it’s actually useful to see how some of this inspired my belief in GenAI. There’s a language called Sanskrit in India. It’s a pretty old language. It’s probably a few thousand years old. And one of the projects that I was doing around four or five years back was to be able to take documents that were actually in Sanskrit and translate them word by word into English. And that’s basically when I realized the power of AI in general and even Emerald models, because I was able to take a very obscure document that was thousands of years old and be able to figure out a word by word translation of that. And I was looking at this and I was going, “Oh wow! This is absolutely transformative.” And now if I look back, I feel like we are now in an inflection point. This is very similar to how when the mobile internet came out, the internet came across or the mobile phones. This is an inflection point, and I do believe that what we are doing right now is going to be transformative in the era of technology, period.
Daniel Newman:
Well, Baskar, I want to thank you so much for taking some time. It’s good to learn a little bit about you personally. And of course, congratulations on so many announcements. By the way, always been a thing with AWS. I have to say, I’ve read your earnings reports for years. There’s always been that part at the end of the report that talks about all the releases each quarter. And I’m always away about how much innovation, how much advancement. The only challenge maybe at times is just how does everyone keep up and make sure that they’re always consuming. But of course, you’ve built a really significant workforce and team to work with all your customers on that. Let’s have you back soon. Let’s make sure that you’re not a one time on The Six Five, and congratulations and have a great rest of your week here.
Baskar Sridharan:
Fantastic. Thank you, both. It’s been awesome to chat with you all. And I’m super excited, as I said, and great. I would love to be back here.
Daniel Newman:
Yeah, we’ll do it again soon. And thank you everybody for tuning in. The Six Five is here. We are on the road at AWS re:
Invent 2024 here in Las Vegas. Hit subscribe. Join us for all of our content coverage here at the event, and of course, all the coverage on The Six Five. We appreciate you being part of our community. But for this episode, it’s time to say goodbye. We’ll see you all later.
MORE VIDEOS

Six Five Live at HPE Discover | Rethinking Hybrid Cloud: Control, Cost, and Flexibility
David Nicholson, Keith Townsend, and Matt Kimball join the Six Five to discuss HPE's advancements in hybrid cloud at HPE Discover 2025, focusing on control, cost, and flexibility. A deep dive into how these initiatives are reshaping enterprise cloud strategies.

The Six Five Pod | EP 265: Will AI Take Your Job? Plus Intel’s Quiet Transformation & AMD’s Opportunity in the AI Chip Race
On episode 265 of The Six Five Pod, Patrick Moorhead and Daniel Newman, hosts of the Six Five podcast, dive into the latest tech news and trends. They discuss HPE Discover highlights, OpenAI's legal battles with Microsoft, and Amazon's AI-driven workforce changes. The hosts debate the ethics of AI companies using web data for training and analyze Intel's strategic shifts under new leadership. They also explore Micron's strong earnings and Nvidia's continued dominance in the AI chip market. Throughout, Patrick and Daniel offer insightful commentary on the rapidly evolving tech landscape, punctuated with their signature banter and industry expertise.
Other Categories
CYBERSECURITY
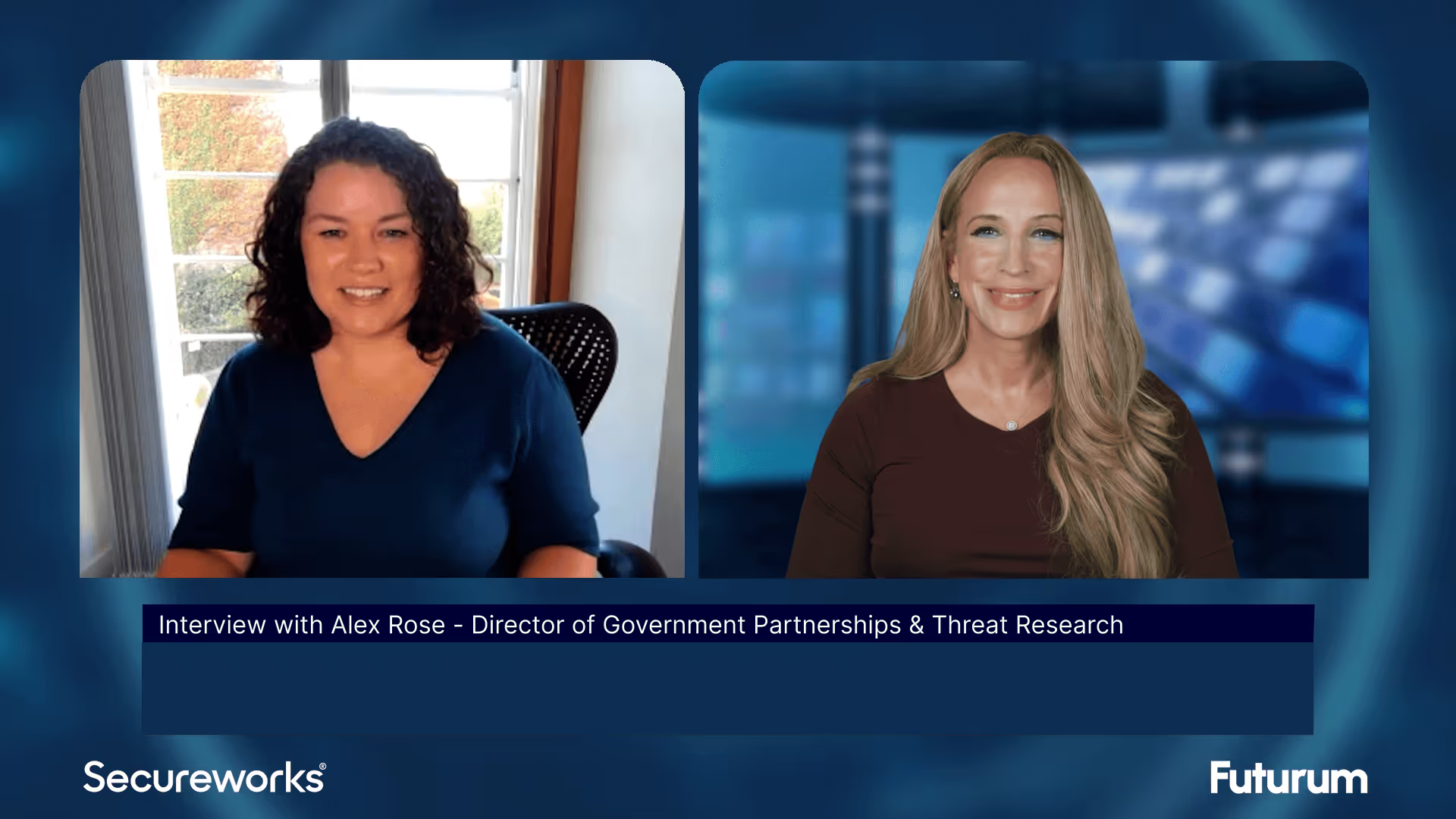
Threat Intelligence: Insights on Cybersecurity from Secureworks
Alex Rose from Secureworks joins Shira Rubinoff on the Cybersphere to share his insights on the critical role of threat intelligence in modern cybersecurity efforts, underscoring the importance of proactive, intelligence-driven defense mechanisms.
quantum

Quantum in Action: Insights and Applications with Matt Kinsella
Quantum is no longer a technology of the future; the quantum opportunity is here now. During this keynote conversation, Infleqtion CEO, Matt Kinsella will explore the latest quantum developments and how organizations can best leverage quantum to their advantage.

Accelerating Breakthrough Quantum Applications with Neutral Atoms
Our planet needs major breakthroughs for a more sustainable future and quantum computing promises to provide a path to new solutions in a variety of industry segments. This talk will explore what it takes for quantum computers to be able to solve these significant computational challenges, and will show that the timeline to addressing valuable applications may be sooner than previously thought.