The Importance of Data Quality and Open Architectures in AI Implementation
Brendan Grady and Sam Pierson from Qlik join host Keith Townsend, sharing their insights on the critical significance of data quality and open AI architectures. They delve into the prerequisites for AI-readiness and Qlik's role in enabling successful AI deployments.
If you were AI, how would you reach your full potential? Check out this episode of Six Five at AWS re:Invent, where host Keith Townsend is joined by Qlik'sBrendan Grady, General Manager, Analytics Business Unit, and Sam Pierson, SVP of Data Business Unit R&D Organization. Learn about the pivotal role of data quality and open architectures in realizing the full potential of AI technologies within organizations.
Deep dive into this ⤵️
The essentiality of a robust data foundation as a precursor to AI adoption and the potential pitfalls in the absence of a solid data groundwork. Identifying the hallmarks of a dataset that is genuinely prepared for AI utilization. The significance of open architectures for AI implementations, including the risks of siloed systems in the evolving landscape of AI technology. Insight into how Qlik is assisting its clients to establish strong data foundations and spearhead successful AI projects, complemented by illustrative examples. Reflections on instances where AI initiatives have faltered and the strategies that could have steered these projects back on course.
Learn more at Qlik and Futurum’s research about AI and Data Infrastructure.
Watch the video below at Six Five Media and be sure to subscribe to our YouTube channel, so you never miss an episode.
Keith Townsend:
All right. Welcome to Six Five On The Road. We’re at AWS re:
Invent 2024, and AWS has some amazing announcements, and we’re going to talk about the ecosystem, the ISVs, this mystical ISV partner, that AWS reference from the keynote we have with us today. Qlik, Brendan and Sam, both data and analytics. You two lead two very important business units within Qlik. So I’m going to start off the question as an AWS all in fanboy. S3 tables, I no longer even need a database, let alone third party services. What value is Qlik bringing in this ecosystem of ISV partners that Matt talked about from the stage?
SamPierson:
Yeah, it’s a great question. I think the biggest thing for us is that this is just yet another technology. It’s yet another avenue for you to store your data. There’s tons of unique stuff that they’re doing in terms of being able to do compaction and the like, but at the end of the day, you still need to be able to get your data out of those sources. You need to be able to transform it. You need to be able to look at the quality and being able to put all that stuff into S3 tables. But it could also be other things, right? You may have other purpose-built systems for AI. You might have other purpose-built systems for business operations. And so you still, at the end of the day, need to be able to bring data into those systems and have all the capabilities around it.
Keith Townsend:
So AWS surprisingly took 20 minutes to get into GenAI on the keynote. Last year, it was GenAI all the time, the whole conference. This year, AWS did a, I think, masterful job of weaving in GenAI as part of the conversation, not just the conversation. Obviously, Qlik is all about data analytics, integrating that. But talk to me in the frame of how AWS framed it. It’s in support of getting business decisions via GenAI. What is the Qlik story with GenAI?
Brendan Grady:
Look, I think as you realize what sort of happened with GenAI, there was this massive hype last year. I mean, we all felt it, and businesses are starting to realize that it’s a piece of the puzzle. It’s not going to solve everything for you. So as we look at it at Qlik, we see generative AI as complementary in enhancing your ability to make decisions. You have the concept of structured data, which is like your financial sales data, things like that, and then bringing in some of that unstructured data, and you combine those things. You can make better decisions more effectively, more quickly, that help you mitigate risk, make money, and save money. That’s really how we see it. It’s augmenting it.
Keith Townsend:
So talk to me about some of the foundational concepts around your data when we’re preparing it for GenAI.
Sam Pierson:
Yeah, so I think everybody’s been able to go out and play with things like ChatGPT, Perplexity, and the like. And I think as an individual consumer, these are super powerful tools, foundational models that have been trained on the open internet. And I think for enterprises who have their own data that’s private, that’s not accessible outside, this is one of the biggest opportunities to be able to take that data that they have in house, which is one of their biggest assets. And then being able to pair that up, not only with your operational data and your dashboards and everything that you’re already doing today, but then to be able to add this other dimension and to have conversation that will end up giving you the right answers. And I think as we’ve talked with our customers, and we’ve talked with some of our early adopters, this could also just be another target at the end of the day.
If you have, again, you’re putting your data into things like cloud data warehouses, you have to do everything around your transformations. You have to monitor your data quality. You have to do a good job of managing that data as it goes into these systems. And we’ve been able to add generative AI. We’ve been able to add the vector databases, the embeddings, as a target very quickly as a result of it. So to us, this is an expansion of what we’re already doing, but generative AI still needs high quality data. It still needs to be correct, because if you have garbage in, the LLMs and the generative AI tools that you’re using on the consumption side are going to get confused and give you the wrong answers.
Brendan Grady:
And if I can add to that, just I think there’s an important piece there. For any of your listeners that have played around with ChatGPT, asked the question and got some really wonky answer back, like I’ve done it, you’ve done it. That just really drives it home. And our own generative AI solution, what we actually have will actually give you an answer of, I don’t know. Which is perplexing to some people and say, well, wait a minute. Your generative AI solution doesn’t know. And we always say, okay, you could have a generative AI solution that just makes it up. Could be wrong, could be off of bad data.
Sam Pierson:
Confidently wrong.
Brendan Grady:
Confidently wrong as well.
Sam Pierson:
Yeah.
Keith Townsend:
You know what? And I think we kind of make AI, especially LLMs, experts in areas that they’re not expert. We transfer this authority to it that doesn’t exist. As a subject matter expert when we’re talking about data analytics, IT infrastructure. If I go somebody with confidence and say that you can run your web server on a Raspberry Pi, they’re going to listen to me, as ridiculous as that statement is, and it is important to have confidence in your data. So let’s talk about that confidence level in your data. This is beyond GenAI. This is about decision making. How do we know from the customer side if the data is really ready for decision making?
Sam Pierson:
Yeah. Well, I think this is not a new problem. The data quality has been a topic for years, and I think a lot of the same dimensions still apply, right? You’ve got completeness, you have timeliness of data, you have data that needs to be updated. Some customers have tried to solve that problem by keeping their data static and not changing, but then it gets out of date, right? It’s stale. Other customers who are trying to keep it continually updated without some sort of a measurement end up with bad data or incomplete data going into that system that they have to go fix. And so having the intelligence on those data pipelines and those data products that at the end of the chain really helps you, I think, have confidence in it. I think we’ve all been there where you’re looking at a dashboard, you might be looking at a sales dashboard, a pipeline, and you’re looking at the dashboard and you say, this does not look right.
There’s no way that this can be right. And frequently, those people who are really in tune with the data, they’re right. And they can go back and they can pinpoint some problem. And so I think this is the same sort of problem that we’ve been dealing with. One of the ways that Qlik and Qlik Talent Cloud has been able to solve this problem is by having the trust score. So quantifying the quality of the data across different lenses. And we’ve then been able to translate this historical trust score for data into an AI trust score. And so we’ve got a dashboard that allows you to measure data, make sure that it’s ready for AI, along with all the other dimensions that have historically been important.
Keith Townsend:
So I’m listening, and one of the I think architecture alarm bells that rings off is when I can get a score from my data, I love the convenience of doing something like that, but the first thing that comes to mind is lock-in. All right, I’ve done this critical and very difficult process of cleaning up my data, preparing my data for AI and other data analytics, but if I put it in a format that I can’t use it across my different systems, where’s the value?
Sam Pierson:
Yeah. Yeah. Well, I think this is a trend that’s repeated itself over time. You had originally all the data lived in Oracle and then Hadoop and Hive and HBase and all the distributed data systems, all the data got distributed, and then you had things like Snowflake and Databricks then that sort of reconsolidated that data, other cloud data warehouses. And I think the trend that we’re seeing now, specific to Iceberg and some of the announcements that AWS has made around S3, but even more generally other players in the market, I think what everyone is coming to the realization is that they need to compete higher up in the stack.
And so by all of these vendors supporting things like Iceberg, OpenTable formats, Parquet files, it’s something that will reduce the friction in terms of the use of that data. It’ll give users more choice. And one of the ways that we’ve leaned into that is in our pipeline product where you’re building your data pipelines, you can actually store data. In the same pipeline, you can store it and you can compute in different areas. You can use different vendors. And so we’ve really tried to lean into giving users choice because ultimately the users and the architecture and the references that they’re trying to make there, it should be them. And being able to avoid that vendor lock in and let the vendors compete on the basis of their own products is I think the best thing for everybody at the end of the day.
Keith Townsend:
I think we’ll be giving our audience a disservice if we didn’t talk about the importance of data and analytics in that integrated experience. I hear the open format conversations, but there’s a value play between the analytics platform and a data platform within Qlik. Talk to me about that value play.
Brendan Grady:
When I think ultimately what it gets down to is, as we think about it at Qlik is as Sam just described, you’re going to have your data all over the place. But one of the things that we’ve always done extremely well is bringing data together in a single pane of glass, regardless of where it is and what source it’s coming from. It’s something that we’ve always been really strong with. We’re going to continue to do that. We also know that it’s only going to expand where people have their data. Everyone would love to think that all their data’s going into S3. That’s just not reality. We all know that, right? We were discussing mainframes on the way up here, and so we at Qlik are always going to respect where that data is, but we are going to put technologies in place that are going to enable leaders and organizations to bring it together easily in one single location where they can actually make decisions off of it. And that’s how we really see the world.
Keith Townsend:
So talk to me about real-world examples of this data foundation. How are customers putting these solutions to practice within their organizations?
Sam Pierson:
Yeah. Well, we’ve got Sharad Kumar, who is one of our field CTOs, did a presentation at the beginning of the week. It was a joint presentation with Accenture, and they’ve talked about the data product concept and effectively this reference architecture of being able to bring together all of your different sources of data, being able to build the pipelines, being able to have the trust score, and then having those curated data sets that are then being able to be provisioned for other parts of the business. And so we see some of our larger customers actually having things like product owner for data products, roles where they’re actually taking requirements from the consumers, being able to quickly assemble those data sets and having the confidence in them, and then being able to provision those into the user’s hands, not only for operational data, but also being able to put those things into a dashboard.
Brendan Grady:
I think a couple really good example that I can think of is Mercedes. The automotive industry is really struggling. Their supply chains got disrupted. Mercedes uses Qlik products to really get their supply chain data and it drives their manufacturing. Then they use the analytics and AI on top of all of that to understand where their gaps are and where they need to go. So Mercedes is probably one of those leading companies that have done that. You also look at large organizations like Siemens, one of the largest companies in the world where they have really put a trusted data foundation and they make decisions around healthcare and where they’re going to put MRI machines and how they’re going to deploy those MRI machines. So it’s not just about, everyone loves to talk about customer turn and retail from an analytics perspective, but companies like Siemens are applying this data foundation combined with AI and analytics so that they can make decisions that are impacting people’s health and livelihoods. It’s really incredible stories.
Keith Townsend:
So not that we haven’t driven the point home, I think we need to put a fine point on this. Where does this go wrong? We’ve talked about using ChatGPT and getting the wrong answer, but do you have an example of when people try to make business decisions off of bad data and the importance of engaging someone like Qlik to clean up the data, organize the data, and be able to make decisions off the data?
Brendan Grady:
So how long do we have? That’s the question. I would strongly recommend that anybody listen to this, just go to Google and just do AI fails. Just do it. It’s very humorous, but it’s also pretty sad. So you look at a company, I will not name the name, obviously, but it’s a company that did hiring, and they went down this path where they were trying to hire the right types of candidates with the right mix of background and the right mix of ethnicities and everything that goes with that. Their data foundation was bad, and they actually recommended not hiring a certain ethnicity. It went wrong because the data was wrong. I can say this one because this one’s pretty public. The Air Canada story, I mean, what happened with Air Canada. Everyone has heard how that data that they had in their system made a recommendation to an agent that would just patently falls and it cost this guy a lot of money, and then it went out on the Twittersphere.
So all of these stories where you hear about these organizations that have tried to put these AI agents in place, and it all started with the data. I mean, one of the largest companies in the world had one that was spewing racist tweets at people. I mean, you heard that story. That was awful, but that, again, that started with bad data that was just highlighted because of the way the model was trained. It just makes it exponentially worse as you get from the data into the way you make decisions. My own experience is writing my own bio, right? Using ChatGPT gave me a great job title, completely wrong. But those are the types of things that you keep finding. And I think if we thought it was challenging with structured data and trying to make sure the financial sales data is correct, now we’re in the wild west of unstructured data. Getting that right and really getting that data foundation right is going to be critical to every single decision that every CEO is going to make in the world.
Keith Townsend:
I really appreciate you two stopping by. This is an important conversation. Data plus analytics equals business decisions. I think it’s an easy thing to write out, but this requires some detailed thought and partnerships to really get right. Every customer that I’ve talked to that’s gone on an AI journey has been frozen at this data point, getting their data ready for analytics and then for the decision-making process. Stay tuned for more coverage from Six Five On The Road here at AWS re:
Invent. We’ll continue to have this conversation around data, around workloads, helping you make better business decisions around your data and IT infrastructure. I’m your host, Keith Townsend.
MORE VIDEOS

Six Five Live at HPE Discover | Rethinking Hybrid Cloud: Control, Cost, and Flexibility
David Nicholson, Keith Townsend, and Matt Kimball join the Six Five to discuss HPE's advancements in hybrid cloud at HPE Discover 2025, focusing on control, cost, and flexibility. A deep dive into how these initiatives are reshaping enterprise cloud strategies.

The Six Five Pod | EP 265: Will AI Take Your Job? Plus Intel’s Quiet Transformation & AMD’s Opportunity in the AI Chip Race
On episode 265 of The Six Five Pod, Patrick Moorhead and Daniel Newman, hosts of the Six Five podcast, dive into the latest tech news and trends. They discuss HPE Discover highlights, OpenAI's legal battles with Microsoft, and Amazon's AI-driven workforce changes. The hosts debate the ethics of AI companies using web data for training and analyze Intel's strategic shifts under new leadership. They also explore Micron's strong earnings and Nvidia's continued dominance in the AI chip market. Throughout, Patrick and Daniel offer insightful commentary on the rapidly evolving tech landscape, punctuated with their signature banter and industry expertise.
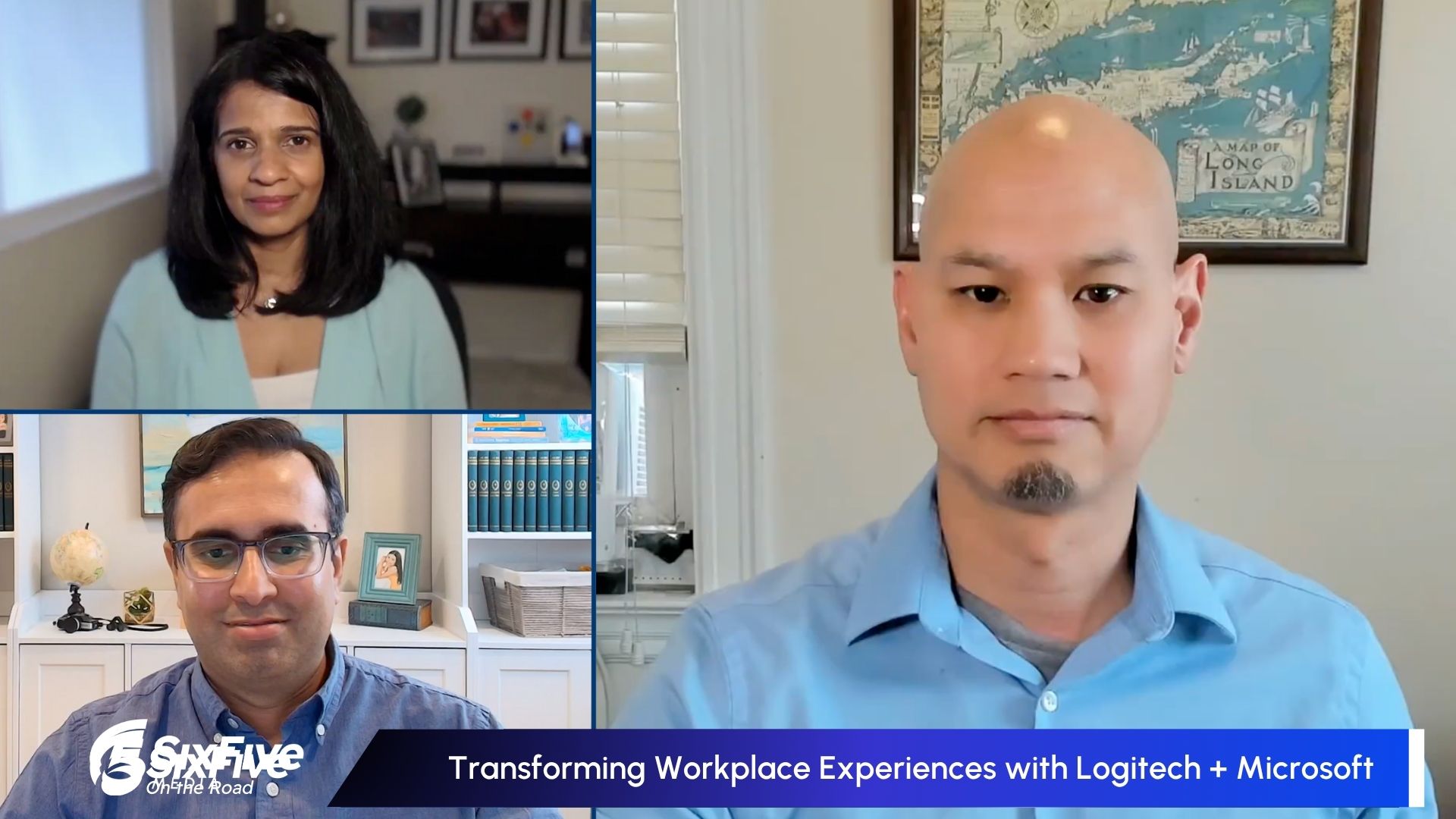
Transforming Workplace Experiences with Logitech + Microsoft - Six Five Media Webcast
In this episode of The Six Five Media webcast, Logitech’s Rishi Kumar and Microsoft’s Sandhya Rao discuss the new meeting technologies announced at their respective events, focusing on Microsoft Copilot, efficiency gains, and BYOD.
Other Categories
CYBERSECURITY
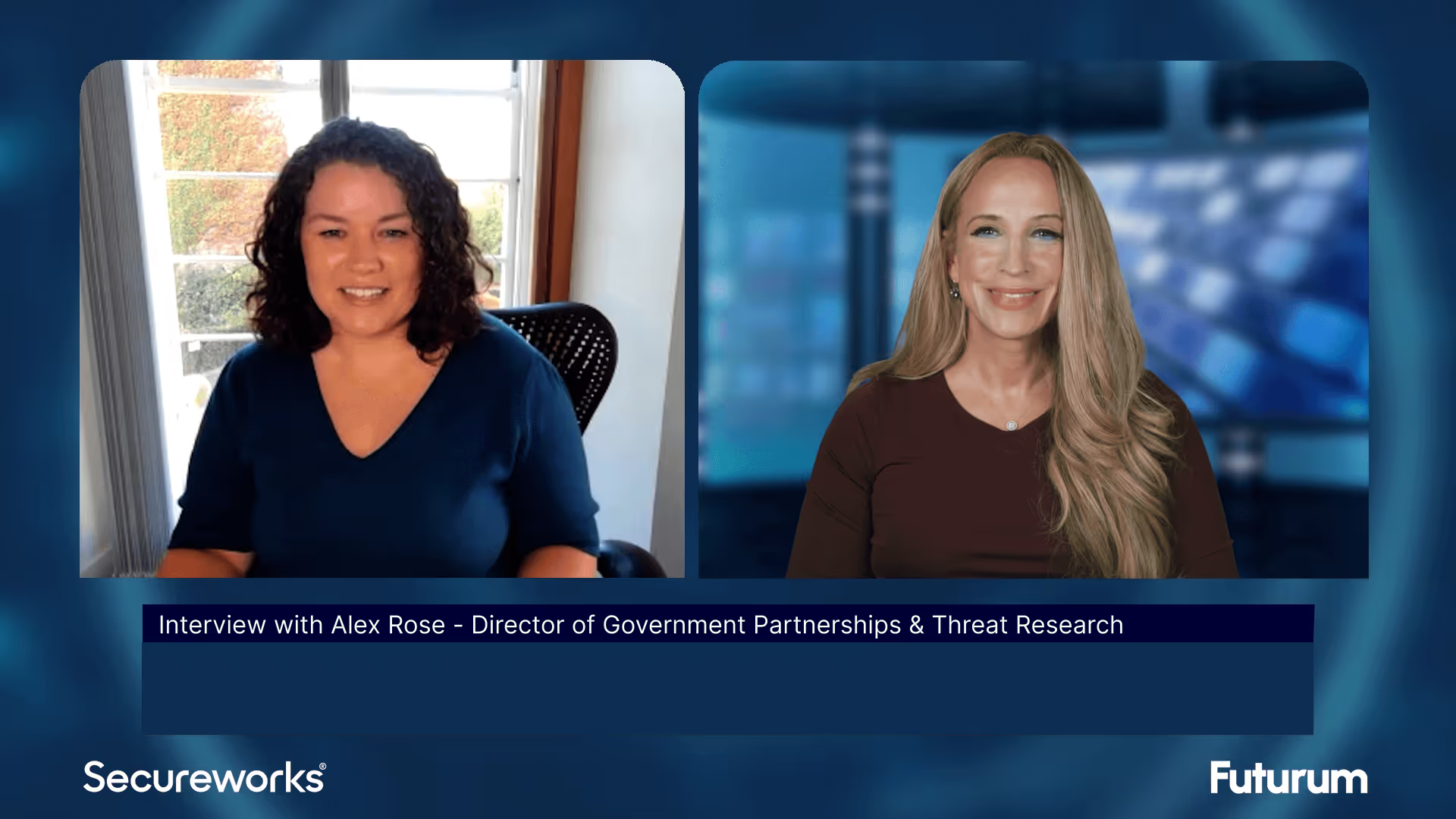
Threat Intelligence: Insights on Cybersecurity from Secureworks
Alex Rose from Secureworks joins Shira Rubinoff on the Cybersphere to share his insights on the critical role of threat intelligence in modern cybersecurity efforts, underscoring the importance of proactive, intelligence-driven defense mechanisms.
quantum

Quantum in Action: Insights and Applications with Matt Kinsella
Quantum is no longer a technology of the future; the quantum opportunity is here now. During this keynote conversation, Infleqtion CEO, Matt Kinsella will explore the latest quantum developments and how organizations can best leverage quantum to their advantage.

Accelerating Breakthrough Quantum Applications with Neutral Atoms
Our planet needs major breakthroughs for a more sustainable future and quantum computing promises to provide a path to new solutions in a variety of industry segments. This talk will explore what it takes for quantum computers to be able to solve these significant computational challenges, and will show that the timeline to addressing valuable applications may be sooner than previously thought.