Maximizing Data Value: The Rising Role of DataOps and Strategic Orchestration
Basil Faruqui, Solutions Marketing Director at BMC, joins Mike Vizard to share his insights on the critical role of DataOps and strategic data orchestration in maximizing data value.
In a rapidly evolving data landscape, organizations must adapt or risk falling behind. Six Five Media Host Mike Vizard connects with BMC's Basil Faruqui, Solutions Marketing Director for Digital Business Automation, to explore how DataOps can be a game-changer. Faruqui shares insights on how businesses can harness the power of DataOps to unlock the full potential of their data and drive digital transformation. Their discussion covers:
- The evolving importance of DataOps in today's data-driven environments
- Strategies for effective data orchestration and automation
- Challenges organizations face in maximizing data value and how to overcome them
- The role of digital transformation in enhancing data operations
- Predictions for the future of data management and operations
Learn more at BMC Software.Watch all of our coverage at Six Five Media at BMC Connect and be sure to subscribe to our YouTube channel so you never miss an episode.
Mike Vizard:
Hello, I'm Mike Vizard and we're back with Six Five Media at the BMC Connect Conference in what I like to call Viva Las Vegas. We're here with my friend Basil. And we're talking about DataOps and how to manage this at scale. Basil, welcome to the show.
Basil Faruqui:
Thank you. Happy to be here.
Mike Vizard:
We've been talking about this DataOps subject all conference, and the issue is there's so much data, there's so many different data types, it's located everywhere you can possibly imagine in formats that people can't handle. How do we attempt to pull all this together in some level of scale that can drive an AI outcome?
Basil Faruqui:
Right. So as you mentioned that the amount of data that the companies are now dealing with is definitely a challenge, but I think what's really bringing DataOps into a sharp focus for companies is that the CEOs and the boards of many companies have realized that the value that can be driven out of data is crucial for their success. So this is no longer an IT initiative, this is a boardroom-sponsored initiative, but the volume of the data, the amount of data practices, gen AI is bringing a whole new world of practices to the world, is creating a challenge at the operational level.
So you've got lots of investment in this space, lots of sponsorship for that investment, but the challenge is with the amount of technologies that are coming out in this space and the amount of data that those technologies are supposed to be handling is a challenge at an operational level. And DataOps really is sort of an acknowledgement by the industry that we need an operational model to be successful if we're going to scale.
Mike Vizard:
We've been tossing around this phrase of data's the new oil for as long as I can remember. It seems like what we've lacked is the pipelines to get the oil to the refinery so we can actually make something useful.
Basil Faruqui:
Yeah. And this is exactly why data pipeline and data pipeline orchestration in particular, because inside the pipeline, if you look at it, it's not one or two platforms. Public cloud, in particular, offers a myriad of solutions specifically focused on data extraction, data ETL, ELT, data warehouses, data lakes. And while there can be a lot of debate about should we be considering ETL versus ELT? Should it be a data lake or a data warehouse? There's one thing that there's no debate about, everybody agrees that in production everything should run in an automated fashion. What the challenge is with so many different technologies from so many different vendors, you really need a single point of control, and really, a strategic approach to this.
Quite frankly, the reason DataOps is a topic now versus before, is because ops was always something people did at the end of a development lifecycle, where now, DataOps... In fact, Gartner's recently released a report which was titled Five Ways of Enhancing Data Engineering Practices, and it was all about ops readiness. So ops has really now become strategic and hence DataOps and variations of it, MLOps, LLMOps, the whole gen AI boom is going to put this whole ops thing on steroids.
Mike Vizard:
Yeah. And part of that means that it's going to be occurring, in some instances, in real time. We've been batching and processing of data as long as we can remember, we all know how to do that. It seems like what's happened now is with gen AI, I need responses in sub-second, and I got to get the right data at the right spot to drive that, and that's not easy.
Basil Faruqui:
Right. So there's definitely a lot of focus on how to get data from its raw form to an insight for the business at the speed of light, which often means realtime. But at the same time, it's not going to be the be-all-end-all for all use cases, you're going to need a strategy which can combine event-driven data movement, some data arrives, a sensor senses something, and then, does take action in many cases where lots of data aggregation is required. So if you think about modern ML models, they can only be trained when you have a large set of data to train it with. So if you think about large-scale data that needs to be fed down our model for training that may not always be in real time.
So if we zoom out and look at it, what I said earlier about data practices, you have bulk extraction, you have time-based extraction, and then, you have change data capture, combine it with streaming, and we're seeing new architectures like Kappa emerge, which are now combining realtime and batch into single streams. So that's part of the challenge, and this is why ops is into focus. How do you not only take the technologies involved in here, but how do you align the data practices along with it in a cohesive manner?
Mike Vizard:
We hear a lot about the phrase retrieval-augmented generation as it relates to AI models, aka RAG. Is that kind of a subset of DataOps as a whole and it's only one piece of the puzzle?
Basil Faruqui:
I think it's definitely a piece of the puzzle because if we think about AI or gen AI and the models, once you've got a well-trained model and it's deployed, it's really a part of a larger data pipeline. You've got data coming from a lot of different sources, that data has to be vetted, it has to be cleaned, sometimes it is transformed, it has to be aggregated, and then, maybe it's going to be fed to a model that's going to analyze and give a recommendation, for example. And the recommendation is often not the last step, what do you do with that recommendation? So let's say you've got an ML model that has predicted customer churn. Well, if you want to send a promotional offer to your customers, the AI model is not the one that's going to send that promotion to the customer, that has to go back to the application layer, perhaps in an ERP or CRM. So when you zoom out and look at this picture, the model as important, and as much focus it has, it's really part of a much larger ecosystem.
Mike Vizard:
All right. We only got about a minute left, but what's that one thing you're seeing customers doing who are successful that you wish everybody else would do?
Basil Faruqui:
So I think the customers that are seeing good success with this, it's a combination of people, process, and technology as always. And from a process perspective, it is to think about ops in a strategic fashion versus something that only happens when something has gone into production. So ops readiness and thinking about orchestration as a strategy is key in sort of defining between success and failure at this point.
Mike Vizard:
All right folks, you heard it here. A wise man once said, failing the plan is planning to fail, it's no different here. Hey Basil, thanks for being on the show.
Basil Faruqui:
Thank you. Appreciate it.
Mike Vizard:
All right, thank you all for watching the latest episode of our adventure here in Las Vegas with Six Five On The Road and our friends at BMC. And please stay tuned for the next set episodes that are coming up right behind this one.
MORE VIDEOS
.jpg)
The Six Five Pod | EP 267: Grok 4, Galaxy Folds, AI Ethics, and Frothy Markets
On episode 267 of The Six Five Pod, hosts Patrick Moorhead and Daniel Newman dive into the latest tech trends and market dynamics. They discuss Samsung's Galaxy Unpacked event, highlighting innovations in foldable phones and smartwatches. The hosts debate the pace of AI development, with Patrick urging caution while Daniel advocates for rapid progress. They analyze the talent war in AI, noting Meta's aggressive hiring strategies. The conversation shifts to market trends, examining the frothy valuations of tech stocks and drawing parallels to the dot-com era.
Other Categories
CYBERSECURITY
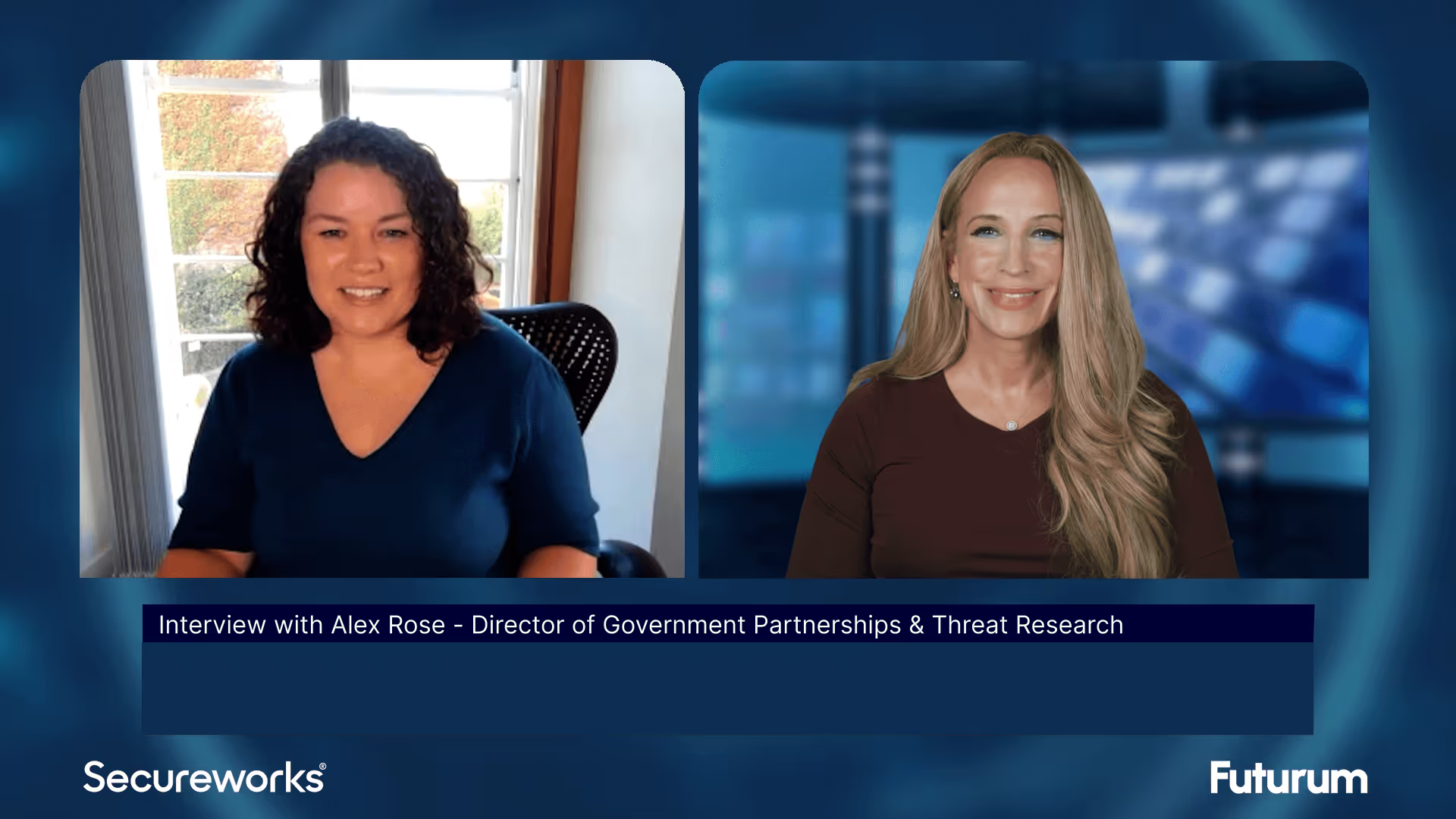
Threat Intelligence: Insights on Cybersecurity from Secureworks
Alex Rose from Secureworks joins Shira Rubinoff on the Cybersphere to share his insights on the critical role of threat intelligence in modern cybersecurity efforts, underscoring the importance of proactive, intelligence-driven defense mechanisms.
QUANTUM

Quantum in Action: Insights and Applications with Matt Kinsella
Quantum is no longer a technology of the future; the quantum opportunity is here now. During this keynote conversation, Infleqtion CEO, Matt Kinsella will explore the latest quantum developments and how organizations can best leverage quantum to their advantage.

Accelerating Breakthrough Quantum Applications with Neutral Atoms
Our planet needs major breakthroughs for a more sustainable future and quantum computing promises to provide a path to new solutions in a variety of industry segments. This talk will explore what it takes for quantum computers to be able to solve these significant computational challenges, and will show that the timeline to addressing valuable applications may be sooner than previously thought.