Accelerating Breakthrough Quantum Applications with Neutral Atoms
Our planet needs major breakthroughs for a more sustainable future and quantum computing promises to provide a path to new solutions in a variety of industry segments. This talk will explore what it takes for quantum computers to be able to solve these significant computational challenges, and will show that the timeline to addressing valuable applications may be sooner than previously thought.
Our planet needs major breakthroughs for a more sustainable future and quantum computing promises to provide a path to new solutions in a variety of industry segments. This talk will explore what it takes for quantum computers to be able to solve these significant computational challenges, and will show that the timeline to addressing valuable applications may be sooner than previously thought. Key takeaways:
- Important sustainability applications can be addressed by quantum computing
- Logical qubits are needed to address valuable applications
- Neutral atom technology is leading the way with surprisingly near-term potential
Robert Hays:
Hi, I am Rob Hays, CEO Atom Computing. We're a 6-year-old quantum computing company based in Boulder, Colorado and Berkeley, California. We've got operations in both. We're building quantum hardware platforms out of the neutral atom modality. So let me tell you a little bit about the platform. So, basically, what you're looking at here is a vacuum system, which is the heart of our system, and in that vacuum system through that tiny black window you see in the bottom right is an array of atoms that we have trapped in optical tweezers. So every individual atom is in an individual laser beam, and with different flashes of light, we're able to manipulate the quantum or the nuclear spin of those atoms, and that becomes the basis of our qubits. And what you're seeing in that grid there is actually through a microscope objective, you're seeing a photograph of individual atoms that are trapped in those optical tweezers.
And this makes really good qubits because we're using a natural material atoms, the qubits are inherent in there, all atoms are identical, so there's no manufacturing defects. We control all these things wirelessly with lasers, and it's generally exponentially scalable with more array or more laser beams in the array. So it's also very compact because atoms are tiny.
And so one of the things that we and our collaborators have been looking at is how do we improve the sustainability of the planet, and specifically, how can quantum computing help us address sustainability not in a marginal or incremental way, but in major breakthroughs. And so there's been a lot of research in this, and this is what's so exciting about quantum computing.
So we ask ourselves, what can we do? Well, what if there were new processes for fertilizer production that reduced carbon emissions and saved a huge amount of the energy consumption worldwide? What if we could bring on orders of magnitude more sustainable resources like wind and solar and batteries to the energy grid and have it be optimized as far as how the power gets routed and more efficient and resilient as a result? What if the fuel efficiency of aircraft could be improved through enhanced modeling and optimized spike dynamics? What if solar cells could be twice as efficient at half the cost through better material simulation and combinatorial optimization? What if food additives and anti-methane vaccines could reduce emissions from cattle through precise molecular simulation?
So these are some of the areas and many more that researchers have been studying around how quantum computing can help, and we've been collaborating with partners on a number of these ourselves. And so for one example is we've been working with the National Renewable Energy Laboratory or NRL on looking at how we could or they have connected our quantum computing into the loop with our supercomputing systems in order to go simulate the digital plan of the electric grid and see how that can be more resilient for basically adapting to new supply of energy coming online, more demand, weather, and different disasters or events that happen in the grid.
But it goes beyond that, energy, agriculture, transportation, smart cities. In fact, McKinsey put out a report last month that said just in these four segments alone, finance, logistics, chemicals, and pharma, there's $2 trillion worth of economic value that can be unlocked by quantum computing through some very specific use cases over the next decade, which is obviously a huge value that we want to go after.
And so what are some of those specific applications or algorithms that are listed here on the right? And we're also mapping to what are the resources required in order to compute some of these different applications? So what we're seeing here is machine learning acceleration, molecular simulations, financial simulation, seismic weight modeling. These are some of the applications that can be unlocked at a production scale around 100 what we call logical qubits. We'll talk more about logical qubits in a second, but that's kind of like the magic number where we have enough resources in the quantum computer to actually produce some of these results that people are looking for in a production environment.
Now, there's other applications and algorithms that are going to require thousands or even tens of thousands or more qubits. They're also going to require what we call deep circuits. So think about as a long deep code or long software programs in order to actually compute some of these applications. And so that raises the question, what more do we need in the physical hardware in order to be able to address some of these applications?
And so, that's what I want to spend a little bit of time diving into, and it's the challenge of deep circuits. And so what we have here is we have an example of a kind of a generic quantum computing circuit. This circuit has four qubits that are represented by the zeros, the four zeros in the brackets on the rows there, and it's a circuit depth of N as represented by the columns there. And want to kind look at how error rates affect the ability to actually compute a circuit of this size.
And so in this case, what we have is we have the qubits have 99.9% fidelity, which means that they would get one error out of every thousand tries. That doesn't sound too bad. That's actually about state of the art for what any of the companies that have produced quantum computers to date have shown. So that's a pretty good error rate, especially by today's standards.
And if you run the first four timed steps through this circuit, you could think of this as lines in a program, as an analogy, we have to take 99.9 and multiply it by itself four times. So 99.9 to the fourth power is 99.6. You go, "Hmm, not so bad." But what if we're running a real circuit that has a real depth that could be much deeper? And so when we look at that and we realize that at 99.9% of physical error or fidelities that we're seeing in the best systems today, after only 500 timed steps through this circuit, we have 60% error rate. And so we might as well flip a coin at that point. What do you need a quantum computer for? That's not a very good error rate. So we need to improve.
So the first thought is, well, what if we improve the fidelity of these qubits by one order of magnitude? So now we're getting four nines, 99.9% fidelity, one error out of every 10,000 tries, and you see that, not surprisingly, you get an order of magnitude better circuit depth for the same error rate. So we move that 60% from 500 lines of code to 5,000, but that's still not sufficient. So what we really need is to get to millions of lines of code to run or circuit depths of millions or even tens of millions to run some of these algorithms, and if you do the math, you're going to see that you actually need to get something on the order of ten, or, sorry, eight nines or ten to the minus eight error rate in order to be able to run these production algorithms that really are going to matter.
And so that same data that I have on the right is represented on the graph of the left. And it just kind of shows you this large gap from where we are today, kind of eight nines fidelity on physical qubits to eight nines fidelity that are required to run meaningful applications. And so now the question is, well, how do we get there? So, again, these are some of the applications and how many logical qubits are required. With the physical qubits that we have today, getting from 99.9 to one order of magnitude better fidelity, physicists believe that they can identify the noise sources, we can drive those out of the system. And with great effort and time and cost, we can actually improve by an order of magnitude, maybe two. Probably not going to get much further than that. And so we're going to need something that leaves this large gap between three or four nines of fidelity and eight or greater nines of fidelity that are going to be required in order to run these applications.
So how do we close that gap? That's where error correction codes come in. So there's been a lot of research in the past about how do we basically map physical qubits to these logical qubits using error correction codes that can actually yield better error rates and so forth and close that gap to logical qubits.
So let's talk about what a logical qubit is. So a logical qubit really just starts with a large number of physical qubits that have a error rate below a certain threshold, probably 99.5, 6, 7 is probably the minimal that most error correction codes can withstand. And then what we do is we basically map a bunch of logical qubits or a few logical qubits on top of a bunch of physical qubits. And so you could think of this as clusters of physical qubits that are connected through an algorithm and some in the hardware that allow them to yield out, by design, a logical qubit that has a much better error rate, lower error rate by design.
And so how we do that is we form these logical qubits by clustering these physical qubits together. We apply algorithms and controls to be able to have those clusters of qubits act together as one logical qubit. And there's a bunch of hardware capabilities that are required in order to make that happen. We need low physical error rates, we need mid-circuit measurement, we need long comparison times, we need to be able to reload atoms. There's a bunch of stuff that actually you don't need to worry about at all. That's our job is to worry about that kind of stuff. But one big takeaway is that in order to get to a large number of logical qubits, we needed an even larger number of physical qubits, and therefore we're going to need the systems to scale. And so having a platform that's scalable to get more and more physical qubits with all of these features and the error correction codes is what the whole game of quantum computing is about in order to realize the value of these applications.
And that's where our platform in neutral atoms is really shining is that we're using these tiny atoms that have this wireless control and proven scalability to really ramp up the number of physical qubits with good fidelity and great coherence times, and then we're working with partners to put novel error correction codes on top of that to yield out the logical qubits that we need.
So here's our roadmap. So our roadmap, we started with our Phoenix prototype that we announced back in 2021. It's a hundred-qubit physical qubit system, and that's been up and running for a number of years and allowed us to do a lot of experimentation and really kind of perfect our technology. We're now working on systems that we announced last year that are 1,225 qubits. These systems will be available more generally to more customers later this year, but those are kind of up and running and what we're currently working on. Our next generation systems will scale by another order of magnitude to 10,000-plus qubits and then again in the next generation 100,000-plus qubits. So we're on a path to deliver an order of magnitude more physical qubits every generation, and this is going to give us a really fast path to logical qubits.
So, in the past, or up until recently, most research on surface codes and other error-correction codes we're requiring something on the order of a thousand-to-one ratio between physical to logical qubits, meaning I'd take a thousand physical qubits, I'd map them down to one logical qubit. And that means that you would need, in order to get 10 logical qubits, you'd need 10,000 physical qubits, and that would say that our next-generation systems would be the first time we start to show off what we call a fault tolerance system. But in more recent times, there's been an advancement in error correction algorithm research, and we're starting to see new codes, new novel codes come online, especially on the neutral atom platform, that are yielding out something like a hundred-to-one ratio on physical to logical qubits, which pulls in the logical qubit roadmap by an entire generation.
So, on the current systems, the current hardware that we have today, we're going to be able to demonstrate something on the order of 10 or more logical qubits on this generation, and we'll, in the very next generation, be able to get to a hundred logical qubits, which is that threshold that everybody's looking for to get over in order to have some of these production applications come to life.
And that's a really exciting, because there's been this proverbial of quantum computing is 10 years out for a long time, but now we're only one generation away from seeing some real meaningful production applications. And in this current generation, we're going to demonstrate all the technologies really required in order to go do that. So the proof of concept and so forth will be kind of imminent, and then we'll have real production out in a very short order in the next couple years, plus or minus.
And so I'd like to just revisit just one application that's really important that we think we can get to when we get to that a hundred logical qubit threshold that we can see on the near horizon now, and this is fertilizer production. So, fertilizer today is produced in large quantities to feed the planet, to feed the fields and grow the plants. It's today produced by one process. Primarily it's called the Haber-Bosch process. This process is a hundred years old. It requires high heat and pressure to produce ammonia, which is one of the primary components of these synthetic fertilizers that we use around the world.
Microsoft put out a paper back in 2016, a great paper that basically studied this problem and how quantum computing could address it. And so you may not know that fertilizer production actually consumes about 2% of the world's energy consumption today. So it's a huge contributor or consumer of that energy, which is great because it feeds the world, but that's a lot of energy. Also produces 420 metric tons of carbon dioxide every year. So if we could reduce this, that would be really meaningful.
Now, again, this Haber Bosch process uses high heat and pressure, but if you look at your compost bin in your garden, you have bacteria and natural processes that are producing the same results and the same nutrients at ambient temperature. They don't require high heat, they don't require energy in, other than just the sun and the natural processes of the bacteria. The problem is we don't understand how that works. And so, it's believed in what this paper studies is that quantum computing actually can provide the right level of simulation at the molecular level in order to really understand what's going on with these bacteria and the natural processes to produce the ammonia, and then hopefully we can reproduce that once we understand how it works at an industrial scale and come up with a new process for producing fertilizer that could significantly reduce the energy consumption required and the carbon dioxide emissions. So that's really exciting use case, and that's just one we see on the near horizon as we get to fall tolerance on our neutral atom platforms.
So with that, thank you. I'm Rob Hays, Atom Computing, and if you'd like to collaborate, reach out, my contact information's on the screen.
MORE VIDEOS
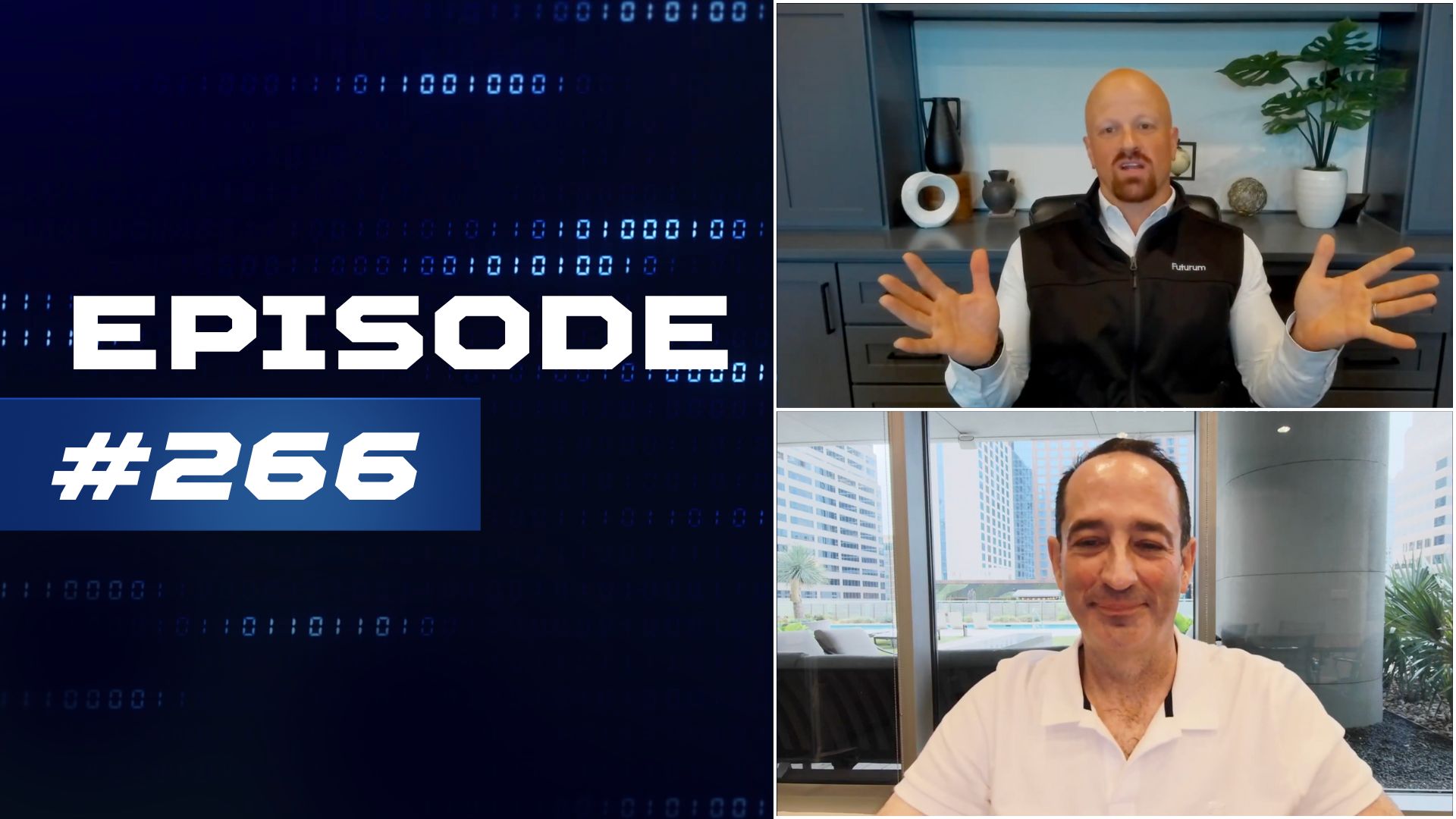
The Six Five Pod | EP 266: Tech Giants' Chess Moves - From Meta's Hiring Flex to Oracle's Cloud Surge
On episode 266 of The Six Five Pod, Patrick Moorhead and Daniel Newman dive into the latest tech news and trends. They discuss OpenAI's talent poaching by Meta, the impact of AI on job markets, and Tesla's robotaxi rollout in Austin. The hosts debate the merits of autonomous vehicles and their potential societal impact. They also analyze recent market movements, including Oracle's $30 billion cloud deal and HPE's acquisition of Juniper Networks. The episode provides insights into the evolving AI landscape, its economic implications, and the resurgence of legacy tech companies in the new era of artificial intelligence and cloud computing.

Six Five Live at HPE Discover | Rethinking Hybrid Cloud: Control, Cost, and Flexibility
David Nicholson, Keith Townsend, and Matt Kimball join the Six Five to discuss HPE's advancements in hybrid cloud at HPE Discover 2025, focusing on control, cost, and flexibility. A deep dive into how these initiatives are reshaping enterprise cloud strategies.
Other Categories
CYBERSECURITY
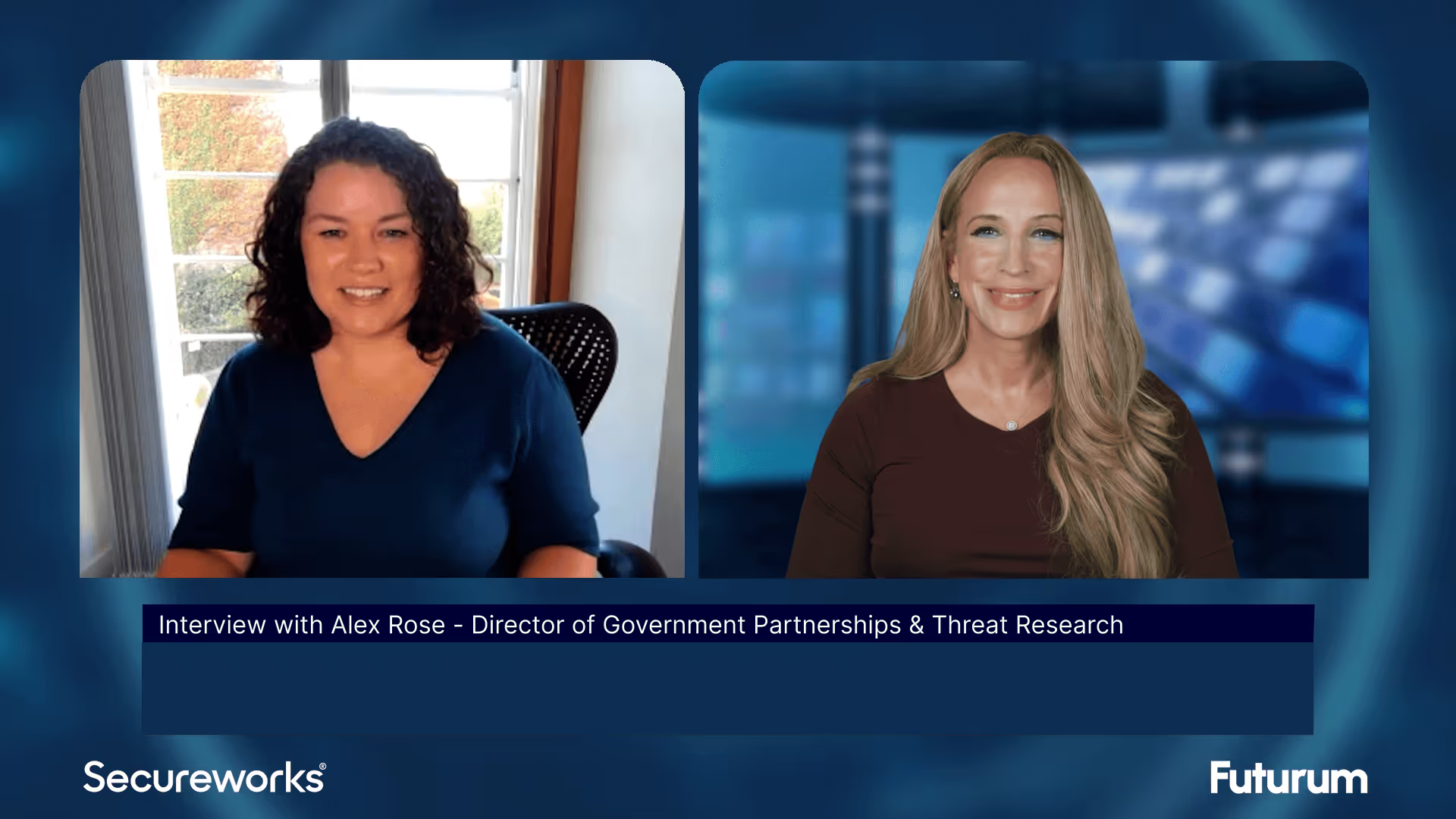
Threat Intelligence: Insights on Cybersecurity from Secureworks
Alex Rose from Secureworks joins Shira Rubinoff on the Cybersphere to share his insights on the critical role of threat intelligence in modern cybersecurity efforts, underscoring the importance of proactive, intelligence-driven defense mechanisms.
QUANTUM

Quantum in Action: Insights and Applications with Matt Kinsella
Quantum is no longer a technology of the future; the quantum opportunity is here now. During this keynote conversation, Infleqtion CEO, Matt Kinsella will explore the latest quantum developments and how organizations can best leverage quantum to their advantage.

Accelerating Breakthrough Quantum Applications with Neutral Atoms
Our planet needs major breakthroughs for a more sustainable future and quantum computing promises to provide a path to new solutions in a variety of industry segments. This talk will explore what it takes for quantum computers to be able to solve these significant computational challenges, and will show that the timeline to addressing valuable applications may be sooner than previously thought.