Groq Builds the World’s Fastest AI Inference Technology
In this session you'll learn: How Groq technology transforms the generative AI infrastructure How Groq’s LPU delivers speed, quality, and energy efficiency What augmenting human capacity means for the future
In this session you'll learn:
- How Groq technology transforms the generative AI infrastructure
- How Groq’s LPU delivers speed, quality, and energy efficiency
- What augmenting human capacity means for the future
Daniel Newman:
Hey everyone. Welcome back to the Six Five Summit, Daniel Newman here, CEO of the Futurum Group. It's day two. We are in one of my favorite tracks. Chips weren't always cool, semiconductors had a period of time where no one wanted to talk about them, but yet I said silicon would eat the world. This goes back to 2019. Didn't even know that we would see the AI trend in the exact form, but I was pretty sure accelerated computing was going to change the world. Today I'm joined here, during day two, by Jonathan Ross, CEO of Groq. Jonathan is no stranger to the Six Five. He's been on for several years because it was several years ago that I began working and we began doing advisory relationship with Groq. And also it was at that time that I said, "This company is doing something pretty fantastic". And that's when I decided to join the cap table, join the ranks of Groq and its leaders, and I had to put that out there because that disclosure was important. But from the time I met you, Jonathan, I knew you were onto something special. I'm glad you're here back at the Six Five. Congratulations on all the success.
Jonathan Ross:
Well, thanks for betting on us. And hello everyone, and really excited to talk a little bit about LPUs and what we're doing.
Daniel Newman:
Yeah, no. And listen, you made it easy. The technology is hard, but the way you described it and the way you have committed to doing something special has been really easy to get behind. It's been incredible. I won't spoil your riches, but you've seen your community grow a little bit. Tell me a little bit about... Give me the last six months or so what's been going on at Groq, because all I can say is it feels like the momentum is building.
Jonathan Ross:
Actually, yeah, six months ago I think is roughly around the time when we first demoed what we had. And then of course demoing is one thing, getting it on our website so everyone could use it was another. And I think we made it available to developers for the first time about 11 months ago. We had a closed beta, we had fewer than 10 developers... Or sorry, 11 weeks ago. And then in 11 weeks we've gone from fewer than 10 developers to over 208,000 developers.
Daniel Newman:
That's a massive number. And I've watched it online, I've shared a few of these demos. Sometimes I'm blown away. I got to step out of the role as advisor, step out the role of investor, and I just watched this from afar and I see you demonstrating these tokens, these side-by-side inference demos. And I'm just like, "Wow".
Jonathan Ross:
That's not even us though. That's the beautiful part, it's the community.
Daniel Newman:
Absolutely. You've basically said, "Here's the race car. By the way, bring your own steering wheel, bring your own arrow, and you put it on the track". And I am watching this and it is just incredible. And I mean, look, generative AI it's definitely a megatrend.
Jonathan Ross:
Yeah.
Daniel Newman:
But Groq is taking a bit of a different approach, Jonathan. I mean you are basically looking at all... Yes, GPUs are a big thing, and there's some different... Accelerators are a big thing. And by the way, you can do some inference on CPUs. It's actually probably still where most inference is done today. That's changing. But talk a bit about Groq's approach to generative AI and how you're thinking about infrastructure to enable this trend to really continue to grow and be consumed and the experience to be good.
Jonathan Ross:
I think one of the big bets that we made early on was that speed was going to matter. I think most of the inference architectures out there, infrastructure that's being built, were all targeted on what's called a batch, which is, "Let's do a single memory read and do as much computation as we can". But that slows things down. Now because inference requires a low cost, you have to come up with something very different if you want to have both speed and cost. We did that. And so by the end of this year, we're actually going to be deploying over 25 million tokens per second. Everyone at Groq carries one of these challenge coins to remind us, and when we get into debate about anything, we just plunk that down on the table and we say, "What helps get this number?" And so that's an amount of tokens per second that a hyperscaler would have; in fact, this is where one of the hyperscalers ended last year. So that's where we're going to be the end of the year.
We also focus a lot on quality. The reason is with language, it's not just about... Unlike an image where if pixels off a little bit, you have to get the exact answer. The difference between should and shall in a legal contract is all the difference in the world. You can't have a slight difference. So we actually came up with this technology we call TruePoint, which is an FP16 numeric but it actually gives you the correct answer, unlike normal floating point.
And so our quality is higher, our speed is higher, and our cost is lower. We're also lower energy. So all these things at the same time typically aren't possible, and we had to trade something off. And what we did was we didn't focus on training, we focused on inference, and that's how we were able to get all of these things at the same time.
Daniel Newman:
And I remember you took a pause there. Because I do remember early on we had some conversations, and I think... And I'm sure deep down the engineer in you still sort of believes you could take the training challenge on, but I think somewhere along the lines you came to the recognition... I'm not putting words in your mouth. I'm just saying, having spent some time with you, I'm sure you have ideas of how we could make that better too. But at some point in time you kind of came to the recognition, Jonathan, that you're like, "Look, we've got something really special here that could handle all these generative outputs".
And I'll tell you, you said two things, or three, or four, but two or three really important things there. The first thing you said is accuracy matters. So these tokens, these responses that you get, a lot of people get really excited, but then they actually verify what they got and you realize that you got a lot of junk, you got a lot of crap in there. Hallucination is sometimes the word that's used, but accuracy has a pretty wide continuum. The second thing is you do talk about the fact that, when you're generating things for business, that the words matter. You should not use a LLM that's going to give you bad results, and you shall pay the piper if you do.
Jonathan Ross:
Every word matters.
Daniel Newman:
Yes. And every word that I get, by the way right now, is not particularly good. Third thing I'd like to double click on, because I'd like to get your take on this, is energy. So I've been reviewing a lot of analysis in the market, and one of the things that has come to a very evident conclusion to me is we are going to run out of power very, very quickly if we continue to deploy AI the way we are deploying AI. These racks, they're not 15 kilowatt, they're 20, they're 25, and it's growing every single day.
Jonathan Ross:
And not seeing 25, it's way higher now. We're seeing 80, 120 kilowatts per rack for GPUs.
Daniel Newman:
Well, what I was going to say is when you actually get to these 72 GPU block... But what I'm saying is it was 15, then it was 20, then it was... So we're progressing. Now to your point 75, 80. How do we deal with this?
Jonathan Ross:
People are building to half a megawatt racks now. That's being designed in preparation for where GPUs are going, and it's unsustainable.
Daniel Newman:
That's the question. What do we do? What do you think we do?
Jonathan Ross:
Also, a single GPU, a GB200 uses twice as much power as your house. Think about that. That means that if you want to deploy a GPU, you have to choose between that GPU or two houses. Yeah. So that's not-
Daniel Newman:
Do you like to keep your house cold in the summer? I like to keep it nice and cool.
Jonathan Ross:
Well, you can keep it nice and warm if you put the GPU in it. So that's not sustainable. And one of the things is for training you need this thing called HBM, high bandwidth memory.
Daniel Newman:
Yep.
Jonathan Ross:
And in terms of financial analysts, what you're looking at in terms of the market, every single bit of HBM that's made is going to be sold, and it's going to be sold for GPUs that are going to be used for training. People are trying to repurpose these for inference. The problem is your bottlenecked on your performance based on that HBM, either because you can't read the weights in fast enough, or because you can't read the sequence length in. So if you have a really long context length for an LLM, it slows down if you're reading it from external memory. If it's already inside the chips, it's instant. And that memory burns power. A GPU burns almost as much energy just reading the weights in the context for a model as we burn in total for the entire end-to-end computation. But then you've also got the system overhead, the networking overhead. So we're heard people talking about hundreds of gigawatts over the next couple years. It's insane amounts of power.
Daniel Newman:
But like you said, it's not sustainable. There's going to have to be an answer for it. Groq, it obviously still requires some power. It's not zero, but it is substantially less, yeah?
Jonathan Ross:
Our 14 nanometer chip is between 1/3 and 1/10 of the power versus the latest GPUs. And the GPUs that are coming out in 2025 versus what we're going to have in 2025, watch this space, we're going to pull ahead further to at least 5x better on energy, but potentially much more.
Daniel Newman:
So for everyone out there. I mean effectively what he's saying to you is you could have either 1/5 as much power used to get the same amount of inference that you would require, right? Is that-
Jonathan Ross:
And this is important, I don't want to ding GPUs just because-
Daniel Newman:
Yeah. Go ahead.
Jonathan Ross:
It's like the amount of power that they use, it's high, but that's a little misleading. What matters is what is the energy per token? How many joules of power are burned to get that word? Because if you are burning something like 3000 joules per second of power, that's actually enough energy to power the amount of force that it takes to lift a adult male off the ground. That's an insane amount of energy. So if you're producing 3000 tokens a second, that's like what an airplane needs to keep you aloft. That's insane. But the amount per GPU doesn't matter as much as that per token, and that's what you should be asking people about.
Daniel Newman:
Yeah. Well, it's a big consideration. It's probably under-discussed. I've said this on a few different conversations here and elsewhere, Jonathan, is that it was interesting when we were pre-generative AI, every technology company was kind of waving this big sustainability flag. All of a sudden we see the gold rush, we see the opportunity, and it's kind of like these are diametrically opposed ideas. Because in order to scale AI, everybody's going to burn more carbon, unless we figure out some really magical solution that right now is not evident. The only thing that is evident is trying to use the most efficient computer architecture for each workload is a good idea, because when you use really inefficient ones you burn a lot more power unnecessarily. Which is I think where your point is, is it's not a bad... Like for the training workloads, GPUs are the right architecture.
Jonathan Ross:
Oh yeah.
Daniel Newman:
For inference though, when you're using this certain architecture, it can be very inefficient for power for what you actually would've needed to do that same thing.
Jonathan Ross:
For every token that you train on, it is more efficient by a multiple to do that on a GPU. So we strongly recommend that when you are doing training you do that on a GPU. If you're doing fine-tuning, if you're doing inference, that works much better on an LPU, and that's where those multiples come in. But I wouldn't be surprised if, at some point, you see someone pull a GPU from a rack somewhere and insert an LPU. But they're not going to then store that GPU, they're going to move it somewhere else where someone's doing training.
Daniel Newman:
All right, Jonathan, I want to put you in the CEO chair here. You've built quite a team, you've made some great expansion, you've grown your developer ecosystem. There is clearly a ton of demand, 208,000 developers today as we talk. Who knows, by the time we actually talk again, where this is going to go. What does the future look like for Groq? What are you focused on? What are you thinking about? What do you want your community thinking about when they think Groq?
Jonathan Ross:
Well, there's been over 80,000 different API keys that have been generated that are active, so that means that that's roughly how many applications. And people have been developing things that need speed and the engagement has been going up. And this is something that not everyone is prepared for when they build something on Groq. We had this one person tweet that they had this story writing app, and when they switched from GPT-4 to Llama 3 70B running on Groq the average engagement screen time or whatever, the time while a user was using it, went from 18 minutes to 31 minutes. So Llama 3 70B is a great model, but it's not quite at the quality of GPT-4. That tells you that speed was the thing that increased that engagement. Well, what does that mean? It actually means you end up needing more compute. So the faster the compute is, the more people want tokens, and the more important it becomes to have that cost be lower, otherwise you're not going to be able to afford it. So as you're building these apps, keep in mind that you first build the app that you want working, but then once you get that speed you're going to be using way more compute than you ever imagined before. And we're here to provide it. 25 million tokens per second. That's our goal by the end of the year.
Daniel Newman:
Here's a one 25 million token pass from Jonathan to all of you. Free. I'm just kidding.
Jonathan Ross:
That'll be one second.
Daniel Newman:
One second, y'all. Listen, this is happening so fast, and Jonathan, I want to congratulate you on the tremendous progress. Something I always say to people is, "Slow at first, then all at once". And sometimes when you're building it can never happen fast enough, but you never quite see exactly when that inflection happens until you're looking back at it. And right now the question is, "Are we looking back at the inflection? Or is that just the beginning and we're still slow, and all at once is still yet ahead?" But either way, it's been amazing to watch the ride. I'm proud to be part of it. I'm telling people out there that this is definitely a technology that you need to put your eyes and your hands on if you have not yet. And Jonathan Ross, CEO of Groq, thanks so much for joining the Six Five Summit.
Jonathan Ross:
Thanks for having me.
Daniel Newman:
All right, everyone. Jonathan Ross was back. Not his first time, hopefully not his last time, but Groq is definitely on fire. Appreciate the chance to talk to all of you. It's day two here at the Six Five Summit. Now I'm going to kick it back to the studio. Stay tuned. Plenty more coming your way.
MORE VIDEOS

Six Five Live at HPE Discover | Rethinking Hybrid Cloud: Control, Cost, and Flexibility
David Nicholson, Keith Townsend, and Matt Kimball join the Six Five to discuss HPE's advancements in hybrid cloud at HPE Discover 2025, focusing on control, cost, and flexibility. A deep dive into how these initiatives are reshaping enterprise cloud strategies.

The Six Five Pod | EP 265: Will AI Take Your Job? Plus Intel’s Quiet Transformation & AMD’s Opportunity in the AI Chip Race
On episode 265 of The Six Five Pod, Patrick Moorhead and Daniel Newman, hosts of the Six Five podcast, dive into the latest tech news and trends. They discuss HPE Discover highlights, OpenAI's legal battles with Microsoft, and Amazon's AI-driven workforce changes. The hosts debate the ethics of AI companies using web data for training and analyze Intel's strategic shifts under new leadership. They also explore Micron's strong earnings and Nvidia's continued dominance in the AI chip market. Throughout, Patrick and Daniel offer insightful commentary on the rapidly evolving tech landscape, punctuated with their signature banter and industry expertise.
Other Categories
CYBERSECURITY
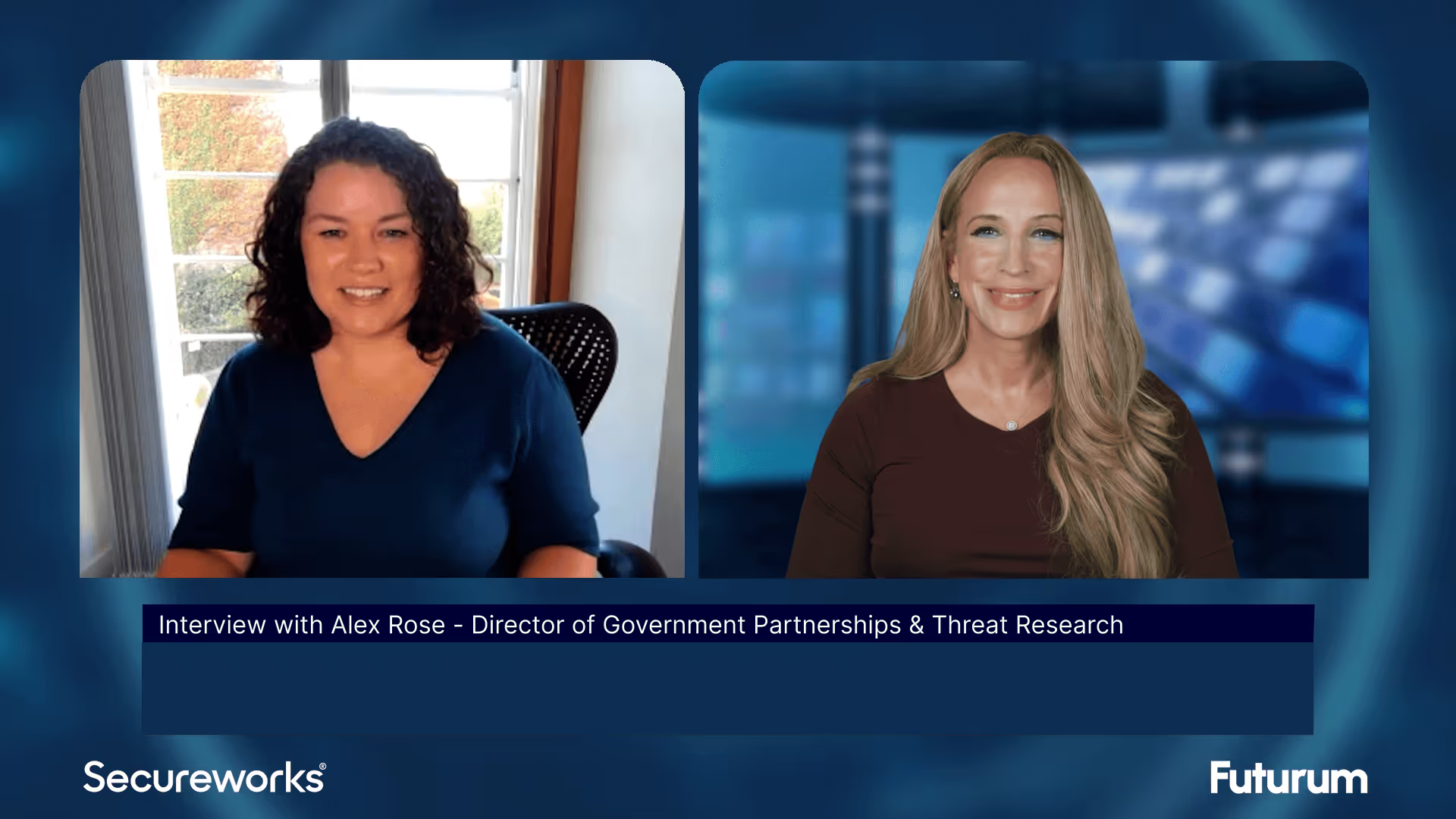
Threat Intelligence: Insights on Cybersecurity from Secureworks
Alex Rose from Secureworks joins Shira Rubinoff on the Cybersphere to share his insights on the critical role of threat intelligence in modern cybersecurity efforts, underscoring the importance of proactive, intelligence-driven defense mechanisms.
quantum

Quantum in Action: Insights and Applications with Matt Kinsella
Quantum is no longer a technology of the future; the quantum opportunity is here now. During this keynote conversation, Infleqtion CEO, Matt Kinsella will explore the latest quantum developments and how organizations can best leverage quantum to their advantage.

Accelerating Breakthrough Quantum Applications with Neutral Atoms
Our planet needs major breakthroughs for a more sustainable future and quantum computing promises to provide a path to new solutions in a variety of industry segments. This talk will explore what it takes for quantum computers to be able to solve these significant computational challenges, and will show that the timeline to addressing valuable applications may be sooner than previously thought.