Advanced AI with Power Edge XE9680 and AMD Instinct MI300X: AI Agents in Action
Delmar Hernandez and Steen Graham, leaders at Dell Technologies and Metrum AI, respectively, delve into the transformative power of AI with the innovative PowerEdge XE9680 and AMD Instinct MI300X, setting new standards for AI agents in action across diverse sectors.
AI agents are no longer a futuristic concept. They're here today, automating tasks, analyzing logs, and even making recommendations. Host David Nicholson is with Dell Technologies' Delmar Hernandez and Metrum AI's Steen Graham for this episode of Six Five On the Road at SC24. Tune in as they discuss how Metrum AI is pioneering the integration of AI agents in industry using the PowerEdge XE9680 with AMD Instinct MI300X.Their discussion covers:
- The innovative features of the PowerEdge XE9680 and AMD Instinct MI300X
- Real-world applications of AI agents in various industries, including healthcare
- The collaboration between Dell Technologies and Metrum AI in advancing AI technology
- Insights into future AI trends and developments
- The importance of hardware and software synergy to maximize AI capabilities
Learn more at Dell Technologies and Metrum AI.Watch the video below at Six Five Media at SC24 and be sure to subscribe to our YouTube channel, so you never miss an episode.
David Nicholson:
Welcome to SC24, the Supercomputing Conference. We are here at the Dell Technologies Compound. I am Dave Nicholson with Six Five On the Road, and we’re going to be talking about some pretty cool AI stuff. I have two incredible gentlemen with me, Delmar Hernandez from Dell Technologies and Steen Graham. And Steen, who are you from?
Steen Graham:
Metrum AI.
David Nicholson:
We’re going to talk all about Metrum AI and what they do, but I want to start with a discussion of a pretty incredible platform that’s just hitting the streets, the PowerEdge XE9680. Did I get that right?
Delmar Hernandez:
That’s right.
David Nicholson:
So tell us about this platform and then we can get into the cool things that you guys have been doing with it, but tell us about this platform.
Delmar Hernandez:
Yeah, so the PowerEdge XE9680 is our flagship AI server. In this case, we’re talking about the AMD Instinct accelerators. This guy has eight accelerators in it. We’ve worked with Metrum over the last few months, actually, to develop quite a few assets on top of this platform. So we supply the hardware, these guys supply the AI solutions that run on top of the hardware.
David Nicholson:
So specifically, these are MI300s. Is that right?
Delmar Hernandez:
Yes.
David Nicholson:
And there are eight in the chassis?
Delmar Hernandez:
Eight of them, yes.
David Nicholson:
So is it fair to say that this PowerEdge server consumes more power than 100 watt light bulb?
Delmar Hernandez:
Yeah, a little bit more. A little bit more.
David Nicholson:
Do they have to be water-cooled or is that very dependent upon how you have these configured?
Delmar Hernandez:
Oh, that’s a great question. So the XE9680 is still air-cooled. This is an air-cooled server, yes. It’s a 6U server, so it’s a little tall, but it’s still air-cooled for sure.
David Nicholson:
Okay. So what are some of the cool things that Metrum and Dell are doing with this? I’m a long-time hardware guy, so I could sit and Delmar and I could talk about this for the rest of the segment about how cool this is, but let’s talk about that next level up. What’s been going on in the world of AI while these platforms have been developed?
Steen Graham:
Yeah, I think one of the things that’s really happened with AI was, where a couple of years ago we were talking about transformer models and the advent of large language models and we were running these single-use large language models in these chatbot applications. And last year we talked about retrieval-augmented generation because we knew that we wanted to supplement those models with accurate retrieved information to put them in a position to not hallucinate and give us accurate information. But over the last nine months, what we’ve seen is the rise of AI agents. And that really perfectly pairs with the platform that the team at Dell has developed because the XE9680, especially with the MI300X accelerators, it gives us the memory footprint to deploy a breadth of software on this. Because it’s no longer just the LLM model, it’s the embeddings model, it’s the VectorDB or the GraphDB, it’s the agentic framework on top of that, and as Delmar alluded to, it’s all of the software that we need for vertical industry solutions.
And so live down here in the booth, we’re showing a telecommunications use case. We’ve got AI agents monitoring telecommunication infrastructure. That requires telco-based OSS feeds, Kafka-based data off the telemetry. We have to give these agents the opportunity to call APIs to get network statistics like drop call rates. And so as we move to this more systematic compound software stack, it really plays to the strength of the XE9680, packed with MI300X accelerators, which give us the breadth of capacity we need and the performance to run these real-world AI applications.
David Nicholson:
So Delmar, I think it’s kind of funny that he said last year. Dude, it was last quarter. There’s no last year anymore. Too much changes month after month. He mentioned memory available. In a fully loaded out 9680, what are we looking at in terms of system memory?
Delmar Hernandez:
So each GPU has 192 gigs, right? So you add that up, that’s 1.5 Terabytes of GPU memory. So like one of the approaches that we took here is how do we showcase the advantage of having that much memory? So working with Metrum, we’ve deployed large language model, like 70 billion parameter models on single GPUs. I can’t remember if we talked about this last time, but I think it was very early, we had not RTS’d this product so that we were a little light on details, but now we can say that we’ve deployed Llama-3.1-70B on a single GPU. We can deploy eight instances of that model on a single server, and then we can train that model on that same server. That’s enabled by the GPU capacity.
David Nicholson:
What’s the footprint of a model? When you say you deployed Llama on a single GPU, I’m an old storage guy. I mean literally, is this like, oh, it’s 100 gig file? Is it a file when you deploy the model?
Steen Graham:
Yeah, and I think-
David Nicholson:
I mean it’s data on the server. You have to have no external connectivity at that point.
Steen Graham:
Yeah, exactly, and I think what Delmar is alluding to is what we can do with the MI300X accelerators is we can fully load a FP or a BF16 precision model in that capacity. You need a little buffer capacity on top of that memory footprint, but that full memory footprint is fully loaded at that point with some buffer capacity to functionally run the model. The cool thing is we couldn’t do that with the other leading GPU on the market because they didn’t have the memory footprint to run that. And that drives the whole TCO story. And you can really accelerate that TCO story when you look at like the Llama recent 405 billion parameter model. You can run that fully loaded, that model takes up all of the memory footprint Delmar just mentioned on an XE9680 with a little buffer enough to run it at that FP16/BF16 precision footprints. And that’s why if you hear like Meta talk about how they’re running MI300X with Llama at 400+B, it’s because that memory footprint allows us to do that on one XE9680 instead of using two, which is a big different.
Now there are different precisions like FP8 so we can take the memory footprint down in FP8. But if you look at some of those solutions we’re deploying downstairs, it’s like, on our medical solution we’re running 70 billion on four of the GPUs, but we’re also running a vision language model for pathology, which we take the skin biopsies and determine if they’re cancerous or not. We’re taking two GPUs to do that. We’re running the embeddings model on another GPU. We’re running the voice model on another GPU. And so you can see how you can easily stretch out that memory footprint to accommodate all this new, I’d say agentic RAG software that needs to be required to do that. And that’s where the TCO story comes in great for the XE9680 with the MI300X accelerators.
David Nicholson:
So talk a little bit more about Metrum in particular. What is Metrum all about? We hear a lot about the collaboration between Dell technologies and Metrum and you’re often talking about some of the forward-looking really cool actual use cases for things built on top of the hardware. What’s Metrum’s story?
Steen Graham:
Yeah, Metrum’s all about high-fidelity, real-world AI and deployed in enterprises and helping enterprises transform their business, whether it’s an AI agent that operates semi-autonomously to support their customers with lower wait times and upsell their customers when they need a higher internet connection bandwidth or it’s a medical solution where we want to enable doctors not to spend 20% of their time typing, which is the CEO of the Cleveland Clinic two weeks ago said the best indicator to a doctor’s productivity is their typing speed because they got so much administrative tasks. And so enabling them with voice capability with the language model to write up the perfect report that they need to write and the right template for their clinic or hospital, incredibly important, and so we’re all about that.
To do that though, one of the other things you need to do to deliver high-fidelity enterprise AI is you need to continuously test all the latest models. And not only on the metrics like throughput and latency and whether they can be observed in a memory footprint on one system or two, because that’s a seven-figure impact. You need to also be able to test the quality of the models based on those domain-specific metrics in development and in production. So we also have the capability to continuously know your AI, whether it’s in development or in production as well. So we care really about measuring the AI so you can know your AI and ultimately grow your business.
David Nicholson:
So Delmar, we have terms and three-letter acronyms, four-letter acronyms coming at us fast and furiously in the world of AI now. One thing that is talked about right now is the concept of an agent or agentic AI, autonomous agents, autonomousity, I just made that up. But if I were to ask you what constitutes an agent in AI, what do you think? And then Steen, I want you to jump in after we hear.
Delmar Hernandez:
So I think this is where I like, so I’ve spent a lot of time digging through logs, platform logs, application logs, operating system logs. I’m sure a lot of our IT people out there have been in the same boat where you’re trying to correlate one log to the other log.
David Nicholson:
Did you find any agents in there?
Delmar Hernandez:
Yeah, no, I was the agent. So you’ve got like a massive set of logs and you’re trying to figure out what broke. So what we’re doing with these AI agents is we’re taking that work out, like we’re solving that problem. The chatbot is actually going in and making these correlations across logs automatically. So I’ll let Steen dig into the details of the application, but we’re real-time streaming application logs from machines that are running in a telco base station.
David Nicholson:
But how does it know what to do? Are you at some point at the beginning, are you telling it this is what I want you to do?
Delmar Hernandez:
Yeah.
David Nicholson:
So you say it does it automatically or automagically, but it’s under your control.
Delmar Hernandez:
Yes, so that’s where the Metrum magic comes in, right? They’re figuring out how to make those correlations on top of the PowerEdge server.
David Nicholson:
Okay, so Metrum magic. Let’s say Metrum magic.
Steen Graham:
Yeah, it’s a great example when we talk about like IT logs. We built a solution where we’re monitoring IT logs, and Delmar does this all the time. When he gets an ethernet port and he gets an error, he knows exactly what he wants to do in that particular case. If you’re managing a bigger infrastructure, you’ve got to do things like do a root cause analysis of what the problem has occurred. And we can tell the agent, hey, here’s what you’re going to do. Look for log failures, not with the human eye, but with your agentic view of the world. And then when you see a log failure, go back into our embeddings model and the vector database and the repository of history and look at what that could be and make a recommendation to the human on what that could be. If you think the AI’s agent, your digital worker’s good enough, you could let them make a recommendation all the way to issuing a work order to repair that system. Hey, let’s pop in a new ethernet port in this particular circumstance.
And so the agents allow us or the way we program agents is we program them the way we would humans do the work. And sometimes that’s like the dull and even sometimes dangerous or dirty jobs as well, but particularly for those dull jobs that we can give the agent that job to do and then the human can be looped in at a later time. It’s incredibly important. For our medical solution that we’re showing, we have a lot more human in the loops on that medical solution, but there is some autonomy on that solution to make determinations.
Delmar Hernandez:
I have a good example. So last night, the servers that were running the demos on live downstairs rebooted, like 2:
00 AM. We came in this morning and they had rebooted and we’re like, okay, what happened? So we went back and I’m emailing the IT guy in our lab like, hey, did you reboot them? Like we have no idea what happened. So I emailed him. He goes and manually checks the logs. We’re looking at the power in the building. All sorts of things to figure out what went wrong. Imagine if with these sorts of solutions, I could just chat with the solution and say, what happened at 2:
00 AM? And then it goes and checks PDU logs, iDRAC logs on the PowerEdge server, application logs running on that server automatically, and it comes back with a response that says this happened.
David Nicholson:
Nunya. It says Nunya. And you say, Nunya? Nunya business.
Delmar Hernandez:
And this can happen in seconds, right? Right Now, this was a 30-minute conversation this morning that we had trying to figure out what happened. This sort of response is like within five to 10 seconds, we get all of these insights, and that’s huge.
David Nicholson:
Do you see having a group of agents, and keep it simple, let’s say you have five of these agents that can perform different tasks. Does that put you in more of a managerial function where you’re orchestrating these agents and having them do things? Does that just make you more sort of horizontally productive from an IT perspective, or does it allow you also, maybe it’s an and/or, I don’t know, does it allow you to focus on higher level tasks? Because that’s always been the pitch. When we talk about increasing productivity, it’s not about you’re going to get to fire 20% of your workforce. It’s, no, no, no. You’re going to make each individual more productive so that they can focus on things that are less tedious, more intellectually stimulating, more likely to contribute greater value to the business. What do you think? It’s kind of a philosophical question. Your experience so far with these agents, what do you think?
Delmar Hernandez:
Yeah, so what I like about it is, like I said, we spent 30 minutes trying to figure out what went wrong and then we solve the problem. Imagine if we could have spent five seconds understanding what happened and then just go solve the problem. So we still have humans coming in and solving the problem, we just understand the problem and then we can go tackle it. So it’s not at a point where it can go solve all of the problems. Of course there are going to be situations where we could maybe have the agent go issue a command to the server and take some action, but there’s still a human that needs to come in and be like, okay, this is the appropriate action, let’s go do this. But they can reach that conclusion faster.
David Nicholson:
I love it.
Steen Graham:
You know, Dave, what that does is that allows everybody a lot more time for those IT administrators or professionals to go build the next supercomputers filled with the next set of digital agents-
David Nicholson:
It never ends.
Steen Graham:
… Because there’s so much work to be done, and I think it’s totally underestimated when you put these really talented people out of the mundane jobs. Now, maybe we can get some more liquid cooling systems up. We can deploy some more servers and build some more agents. And I think the demographics of the globe aren’t great right now. We’re going to need some of these digital workers to help us because people may not want to do these dull, dirty, and dangerous jobs going forward.
David Nicholson:
Well, for myself, Dave Nicholson, from Six Five On the Road, Delmar from Dell, and Steen, father of the Borg as he will be known at some point in time, it’s a great conversation to have when we’re talking about actually finding positive ROI out of AI. You could look up and down the aisles here and you can see all sorts of examples of amazing hardware, but people are asking the question, what are the billions and trillions of dollars actually going towards? This is a great example, what the two of you have put together is a great example of that. Thanks so much for being here for the conversation. Stay tuned for more exciting action from SC24.
MORE VIDEOS
.jpg)
The Six Five Pod | EP 267: Grok 4, Galaxy Folds, AI Ethics, and Frothy Markets
On episode 267 of The Six Five Pod, hosts Patrick Moorhead and Daniel Newman dive into the latest tech trends and market dynamics. They discuss Samsung's Galaxy Unpacked event, highlighting innovations in foldable phones and smartwatches. The hosts debate the pace of AI development, with Patrick urging caution while Daniel advocates for rapid progress. They analyze the talent war in AI, noting Meta's aggressive hiring strategies. The conversation shifts to market trends, examining the frothy valuations of tech stocks and drawing parallels to the dot-com era.
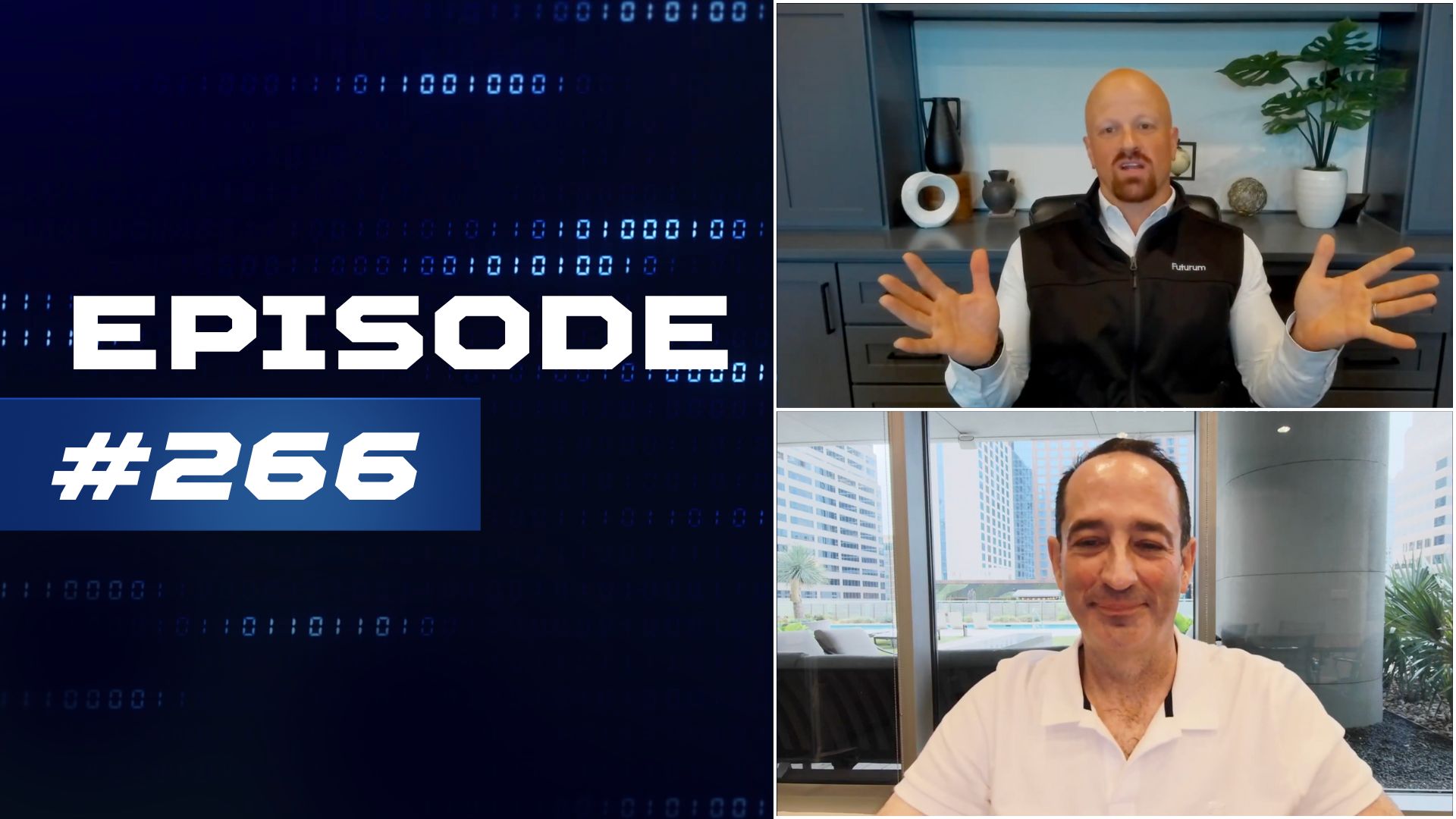
The Six Five Pod | EP 266: Tech Giants' Chess Moves - From Meta's Hiring Flex to Oracle's Cloud Surge
On episode 266 of The Six Five Pod, Patrick Moorhead and Daniel Newman dive into the latest tech news and trends. They discuss OpenAI's talent poaching by Meta, the impact of AI on job markets, and Tesla's robotaxi rollout in Austin. The hosts debate the merits of autonomous vehicles and their potential societal impact. They also analyze recent market movements, including Oracle's $30 billion cloud deal and HPE's acquisition of Juniper Networks. The episode provides insights into the evolving AI landscape, its economic implications, and the resurgence of legacy tech companies in the new era of artificial intelligence and cloud computing.
Other Categories
CYBERSECURITY
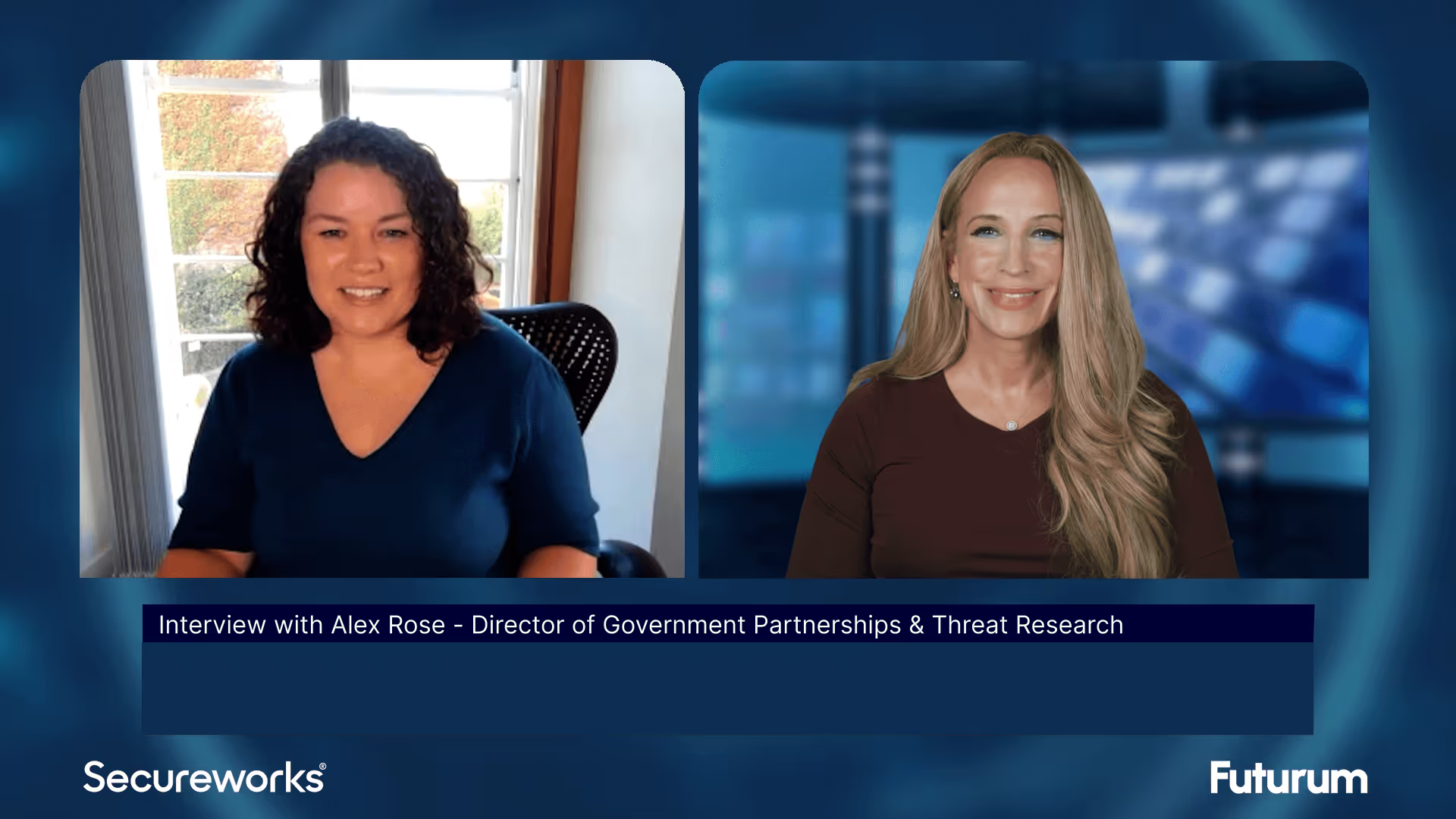
Threat Intelligence: Insights on Cybersecurity from Secureworks
Alex Rose from Secureworks joins Shira Rubinoff on the Cybersphere to share his insights on the critical role of threat intelligence in modern cybersecurity efforts, underscoring the importance of proactive, intelligence-driven defense mechanisms.
QUANTUM

Quantum in Action: Insights and Applications with Matt Kinsella
Quantum is no longer a technology of the future; the quantum opportunity is here now. During this keynote conversation, Infleqtion CEO, Matt Kinsella will explore the latest quantum developments and how organizations can best leverage quantum to their advantage.

Accelerating Breakthrough Quantum Applications with Neutral Atoms
Our planet needs major breakthroughs for a more sustainable future and quantum computing promises to provide a path to new solutions in a variety of industry segments. This talk will explore what it takes for quantum computers to be able to solve these significant computational challenges, and will show that the timeline to addressing valuable applications may be sooner than previously thought.